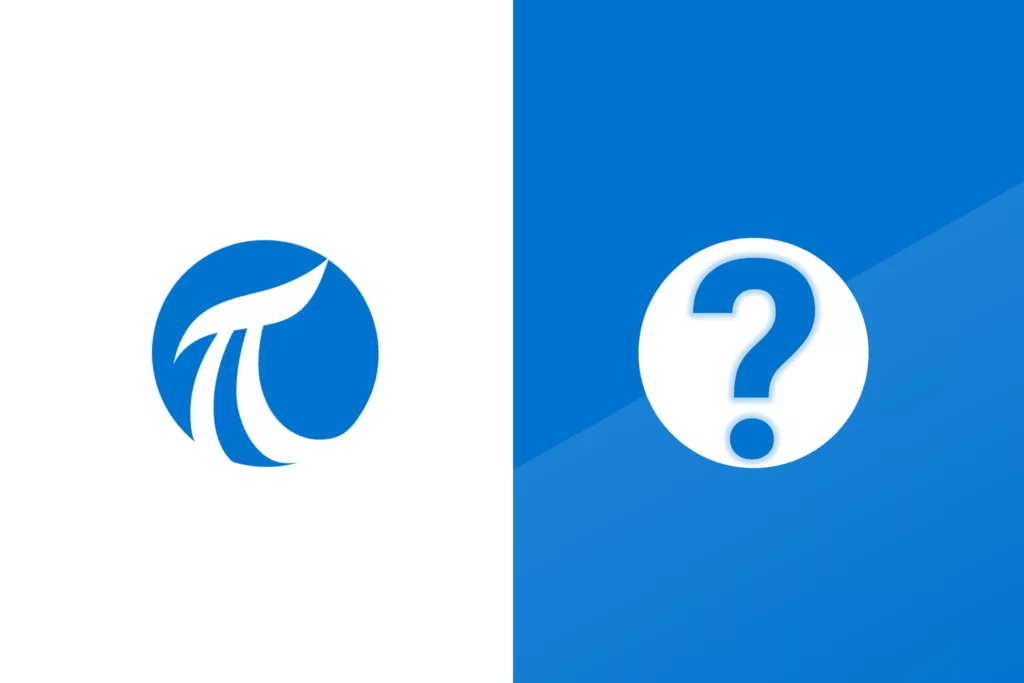
Best Pi Vision Alternatives [2024]
When it comes to process data visualization and analysis, PI Vision is a top choice ...
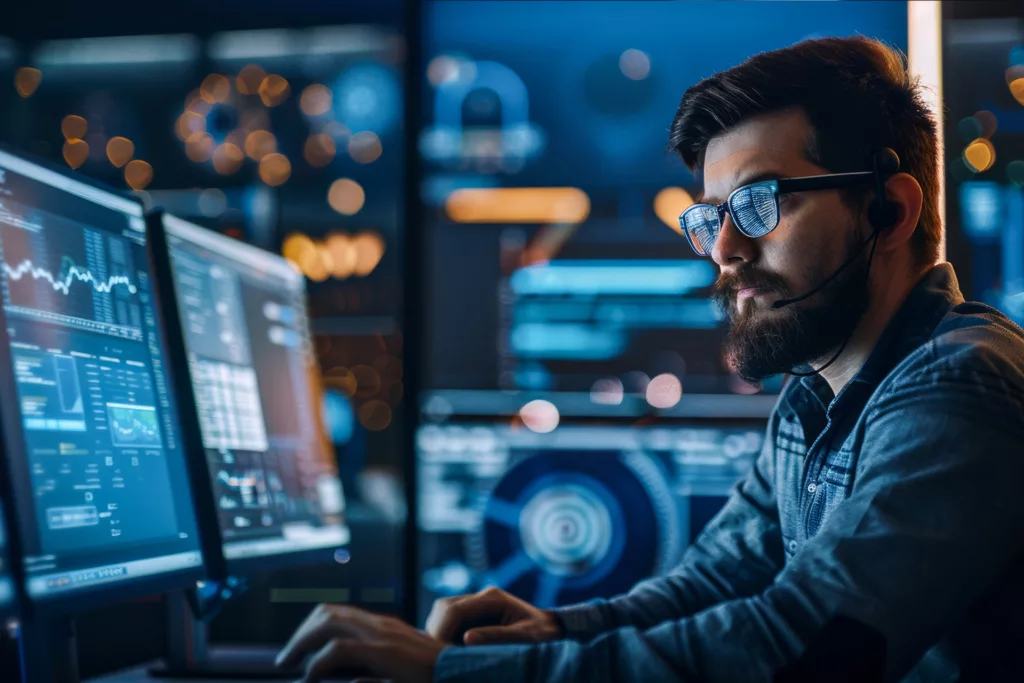
Real-Time Manufacturing Dashboards: Setup, Importance, and Benefits
In this blog, explore what real-time manufacturing dashboards are, why they are crucial, and how ...
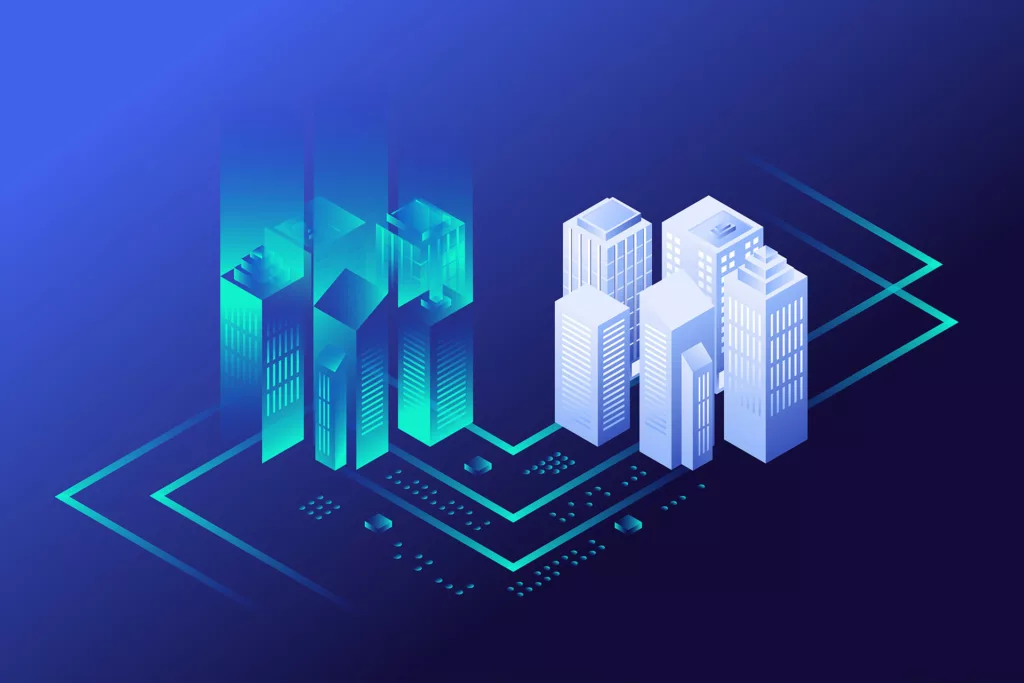
Digital Twins in Manufacturing Explained: Key Advantages and Implementation Insights
Uncover how a digital twin in manufacturing can revolutionize your production processes. Learn how digital ...
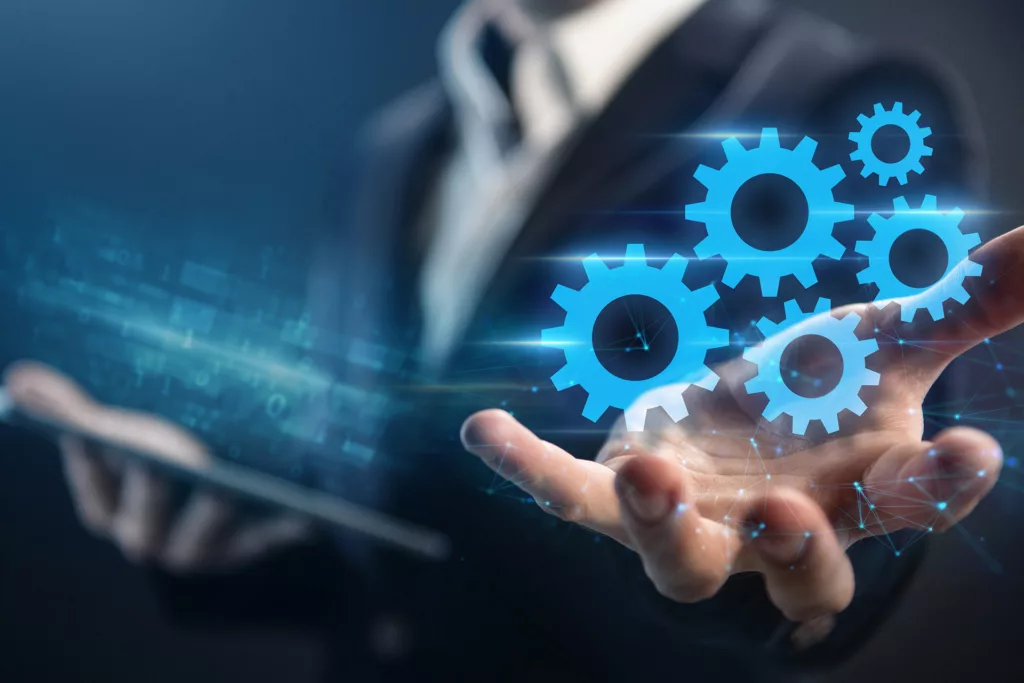
Manufacturing Process Optimization Strategies
Learn about manufacturing process optimization strategies that can boost your output when paired with the ...
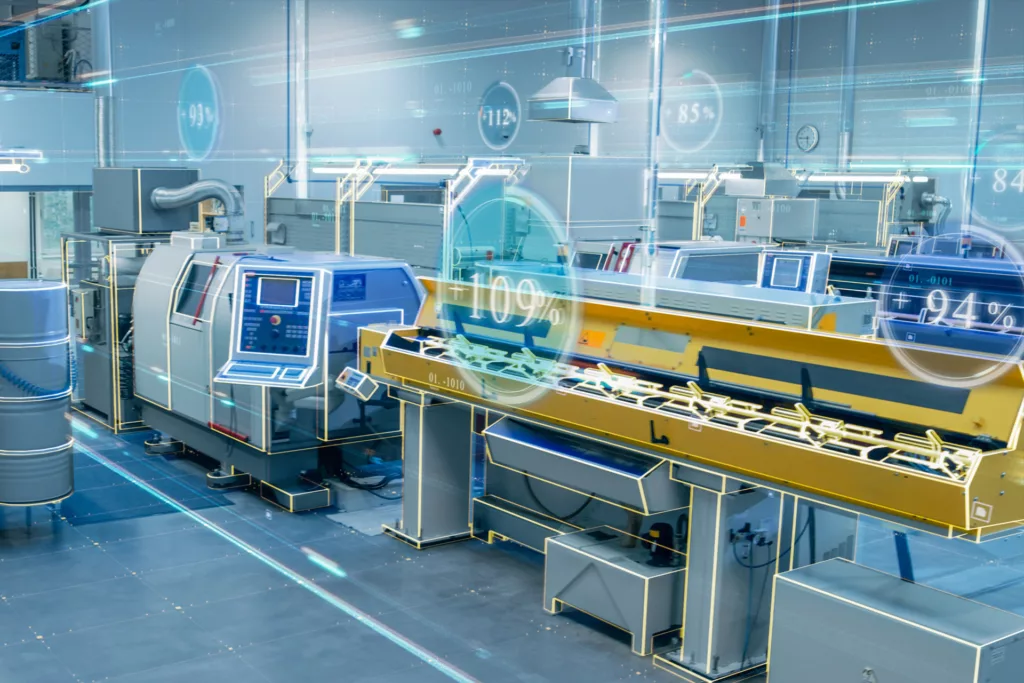
How to Improve Manufacturing Efficiency in 4 Steps
This blog explores four strategic steps to improve manufacturing efficiency: engaging workers, harnessing data intelligence, ...
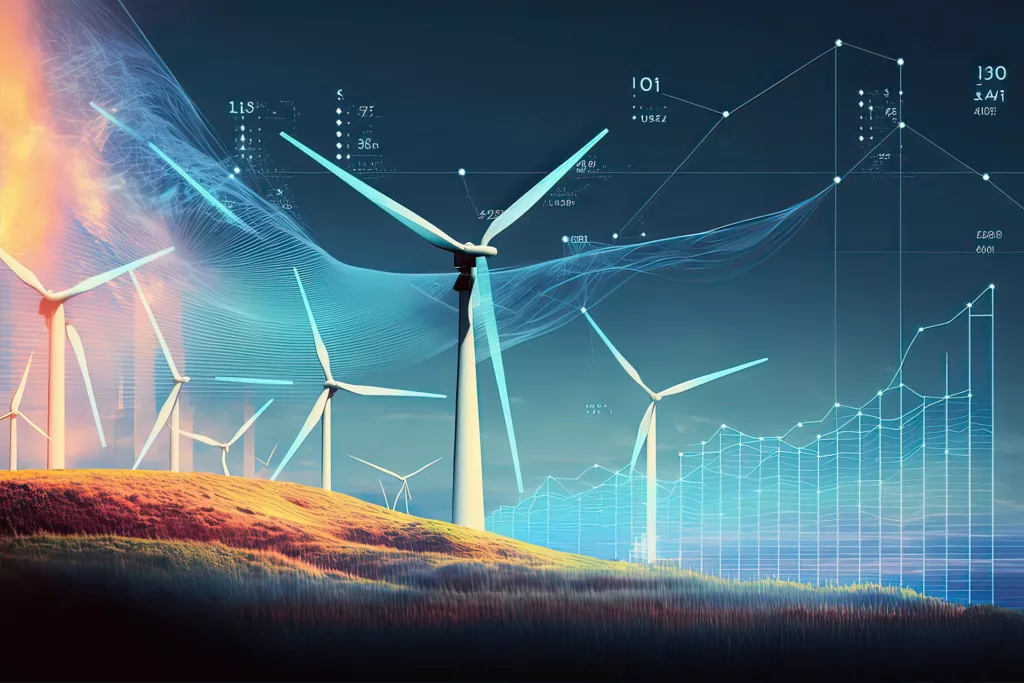
Industrial Energy Management: Track Usage and Gain Efficiency
Learn how to manage eneragy at your site, a strategy that’s becoming increasingly vital for ...
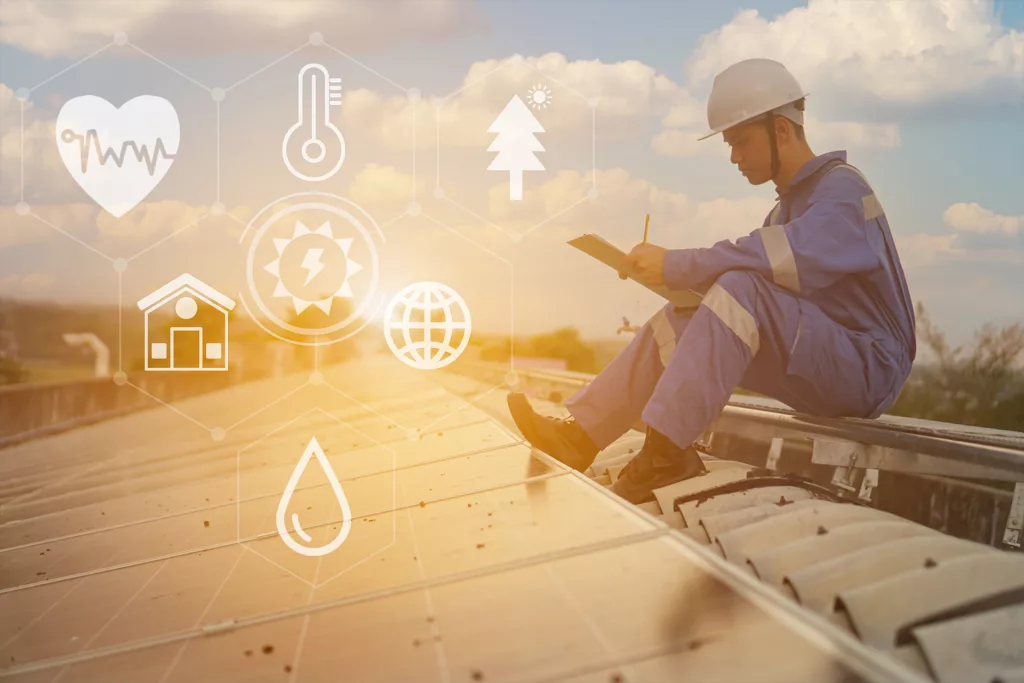
What is Industrial Environmental Monitoring and How to Do It
This blog explores the essential aspects of Industrial Environmental Monitoring, emphasizing its role in promoting ...
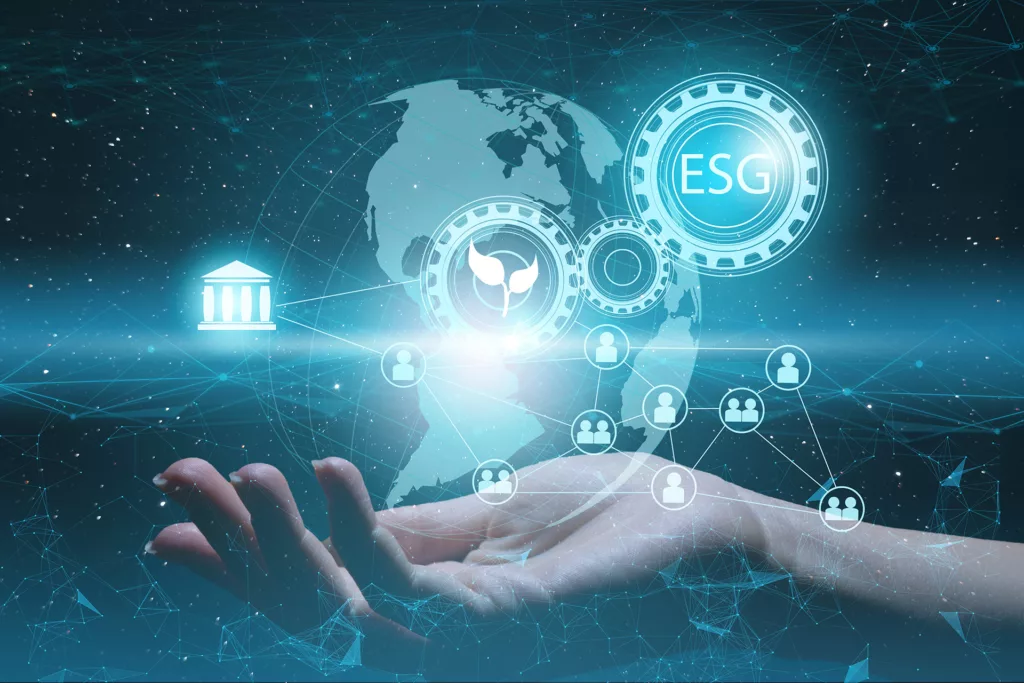
Optimizing ESG Compliance with Environmental Data Software
As businesses continue to adjust with the demands of enviornmental data in ESG compliance, understanding ...
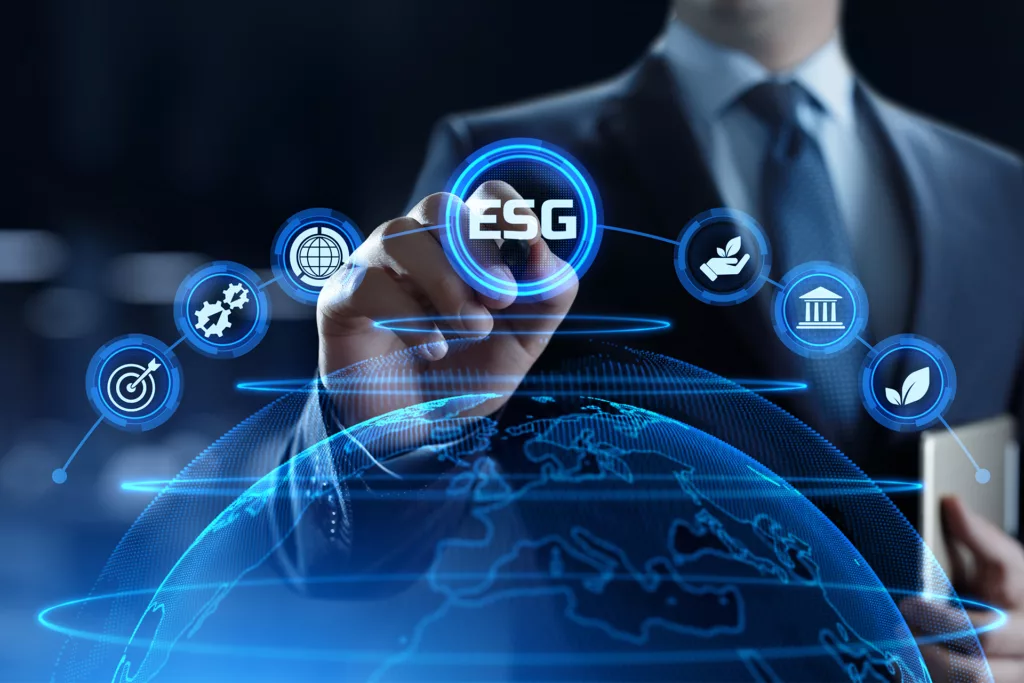
ESG Data Analytics is a Game-Changer for Manufacturing
In this blog post we will explore ESG data analytics, how it pertains to manufacturing ...
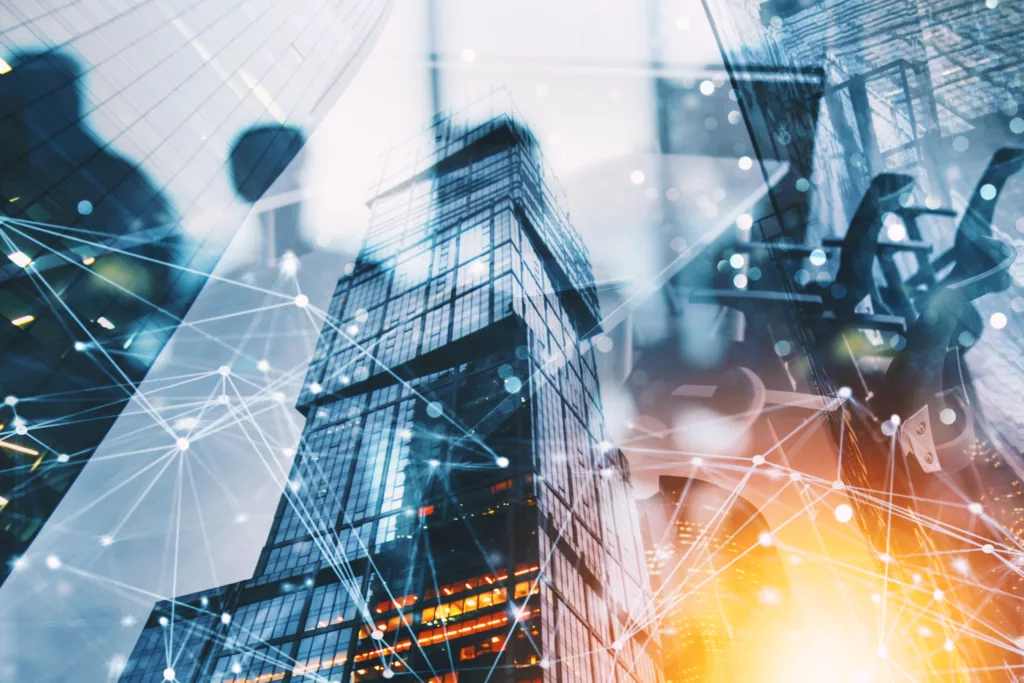
Discussing FactoryTalk VantagePoint Replacements
As the impending discontinuation date of Rockwell Automation’s VantagePoint draws near, manufacturers find themselves presented ...
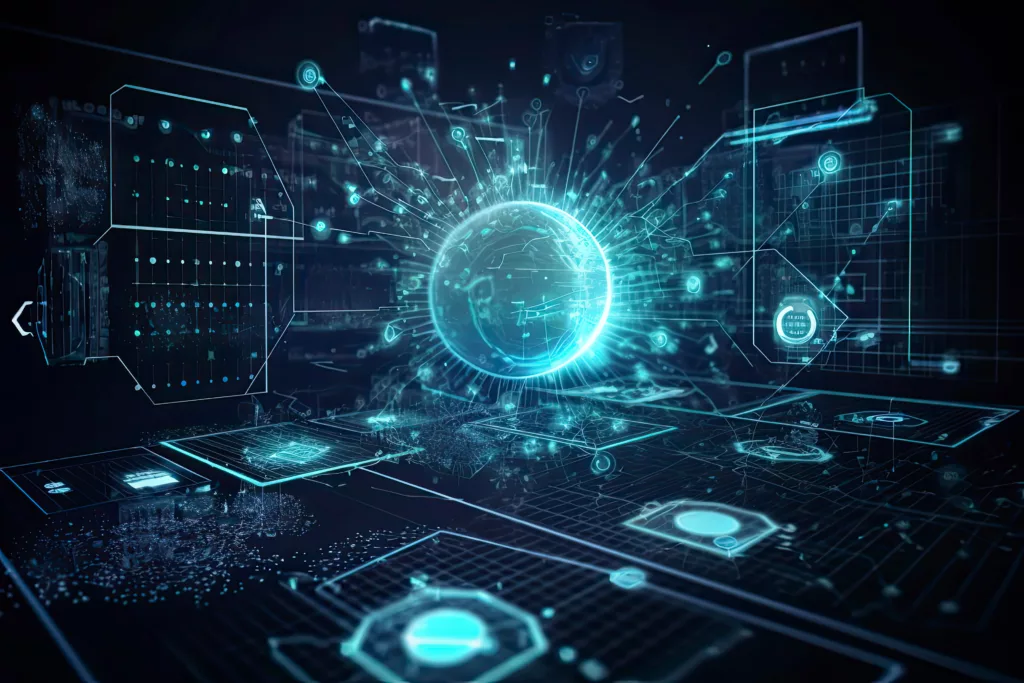
dataPARC Wins 2023 Breakthrough Product Award for its Cutting Edge Data Historian
Nashville, TN – dataPARC has been honored with the prestigious 2023 Breakthrough Product Award, as ...
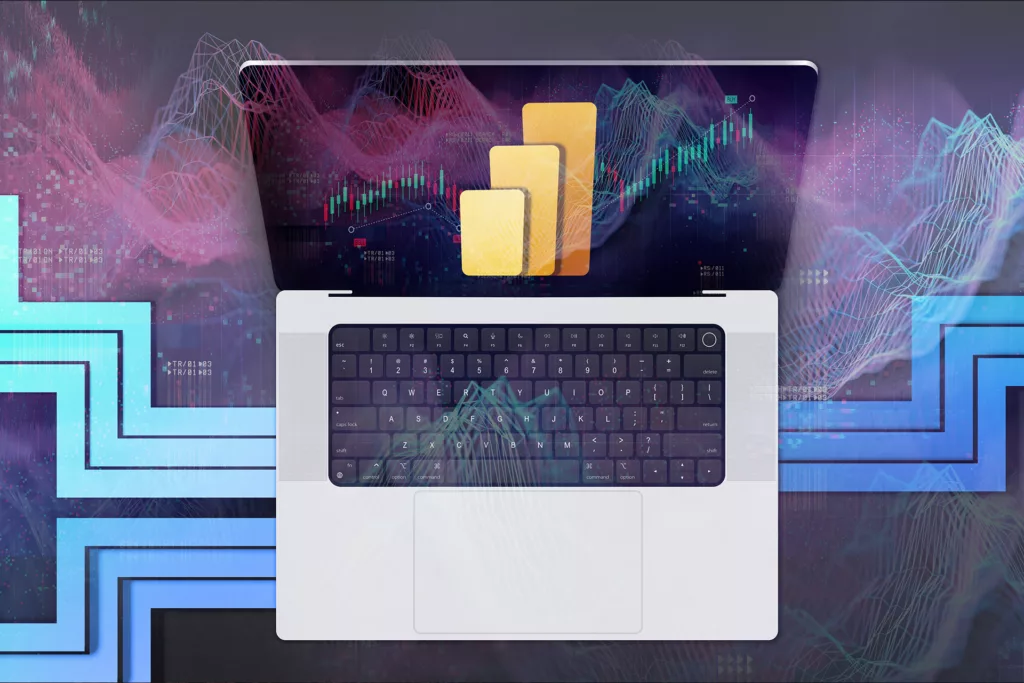
Leverage Power BI Production Dashboards for Real-Time Insights
In this blog, we’ll explore how manufacturing data can be incoprorated into Power BI production ...
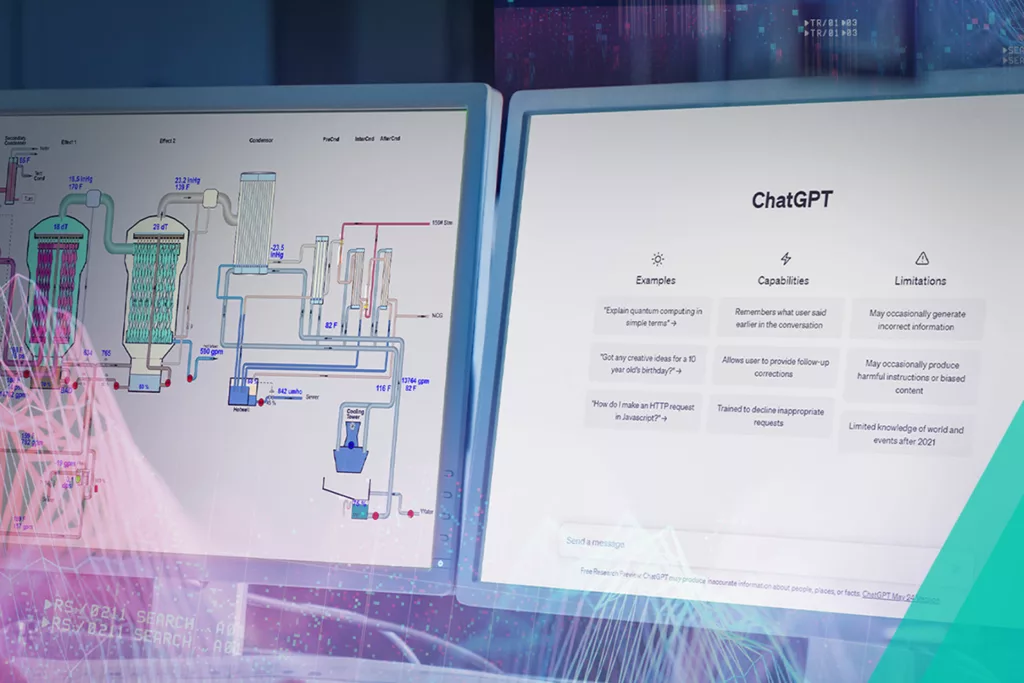
ChatGPT for Engineers: Transforming Graphic Coding from Idea to Implementation
In this blog, we’ll explore how engineers can utilzie ChatGPT to generate code, specifically XMAL ...
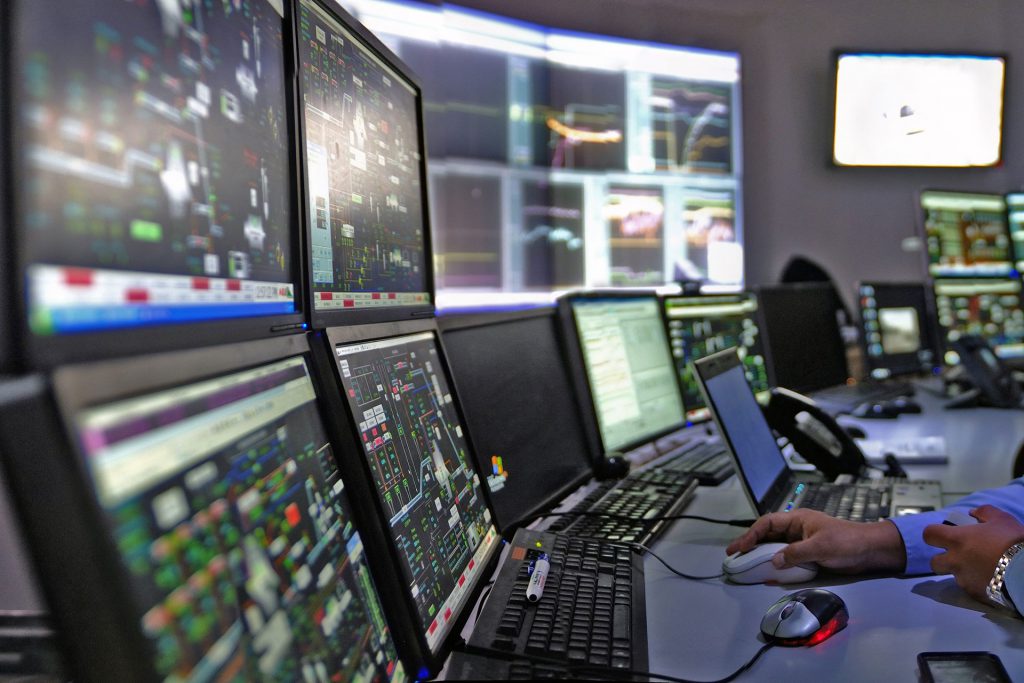
Control Station and dataPARC Announce Partnership to Improve Data Utilization and Plant Efficiency
By integrating dataPARC’s cutting-edge historian with Control Station’s PlantESP Software, plants can now seamlessly optimize ...
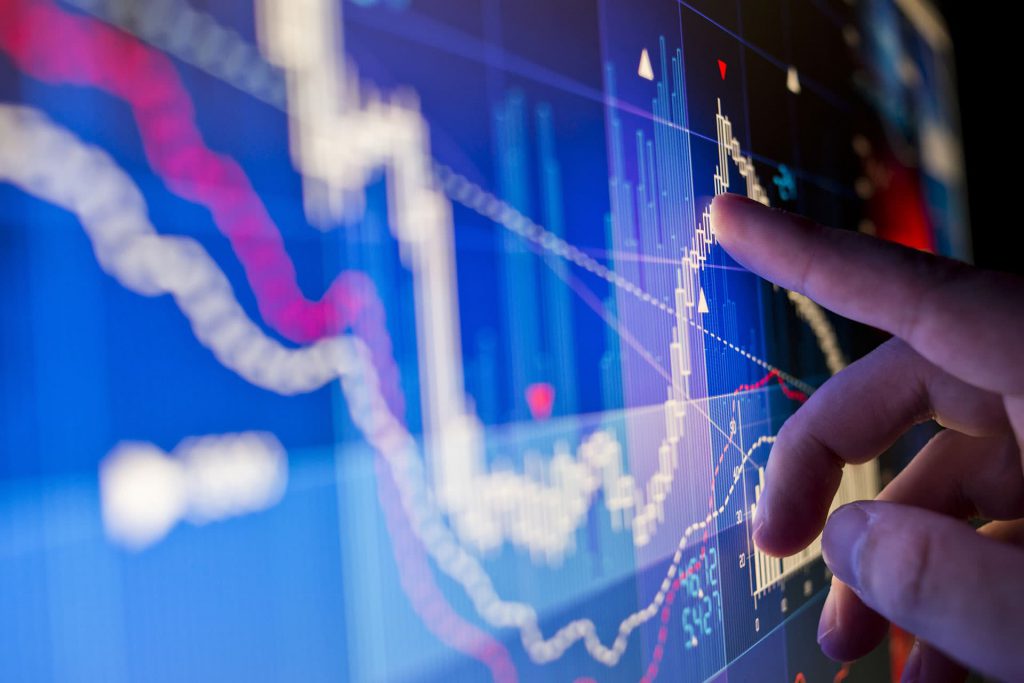
Replacing GE Digital’s Operations Hub: Exploring Innovative Alternatives
In this blog, find a replacement for GE Digital’s Operations Hub that will fit your ...
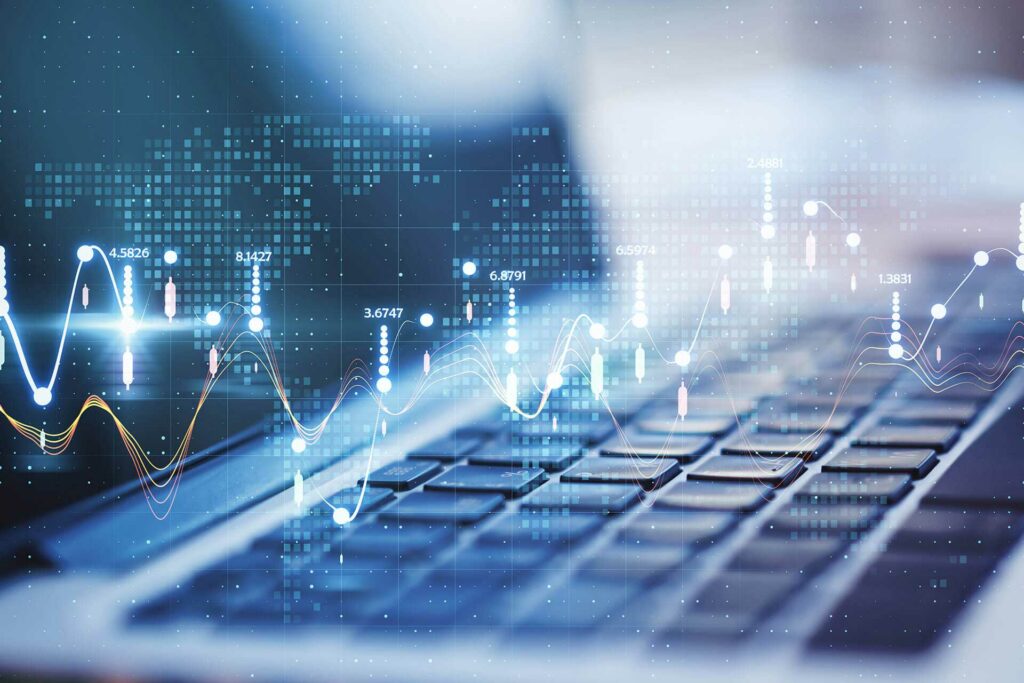
Top Data Visualization Tools for aspenONE Process Explorer Replacement
In the dynamic landscape of process industries, staying ahead of the competition requires robust data ...
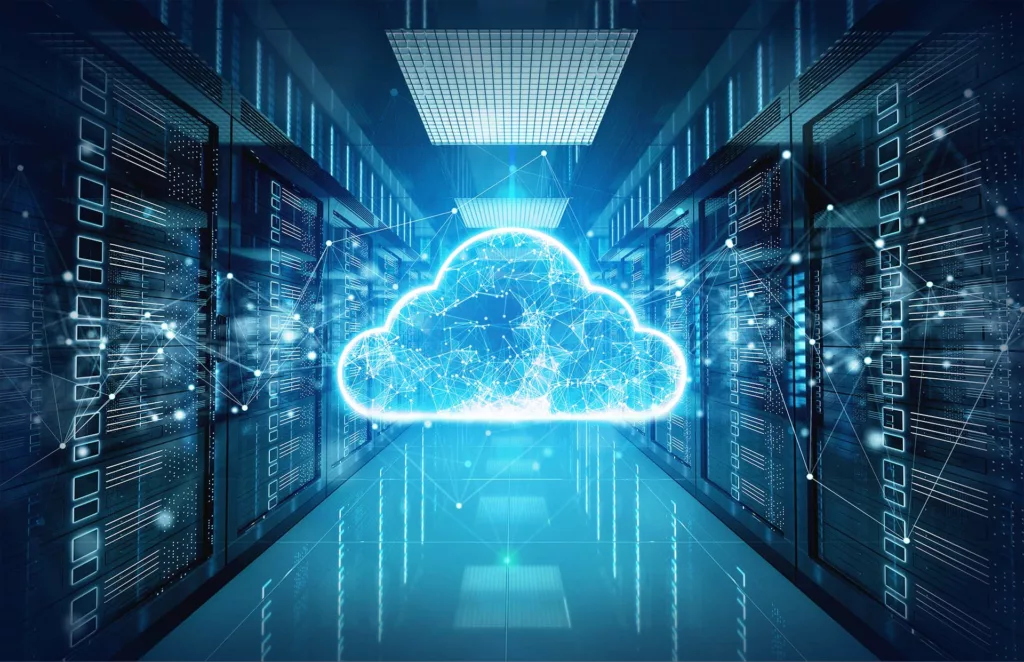
Find the Right Fit: Choosing Between Cloud and On-Premises Data Historians
This blog compares on-premises and cloud data historians. It examines the benefits and drawbacks of ...
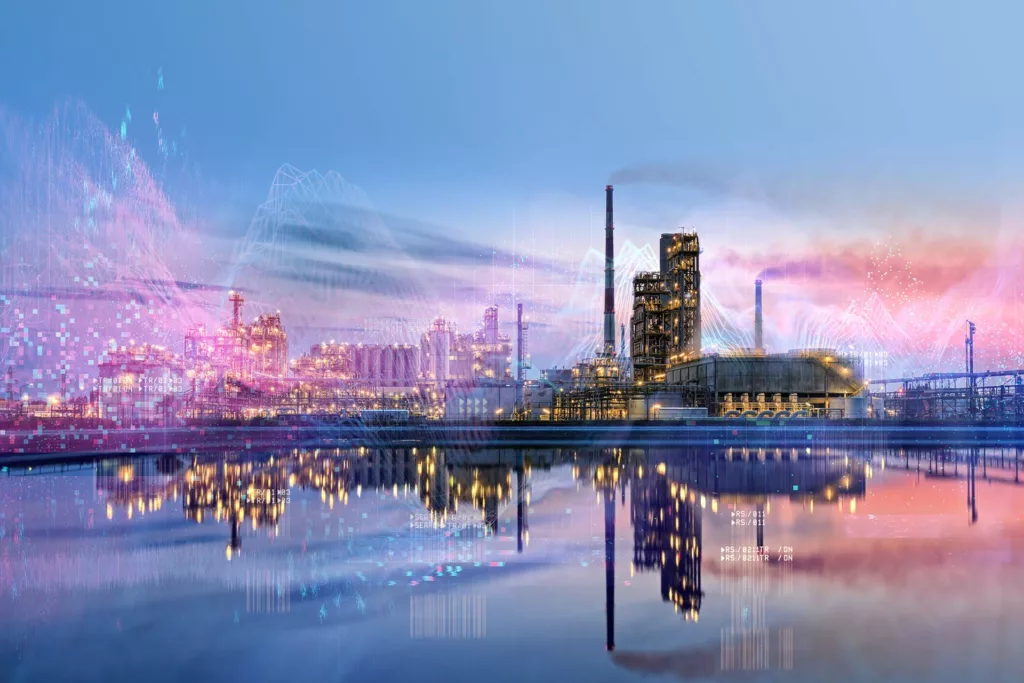
Exploring Alternatives to Honeywell Uniformance for Data Visualization
When it comes to finding alternatives to Honeywell Uniformance, organizations must carefully evaluate potential solutions ...
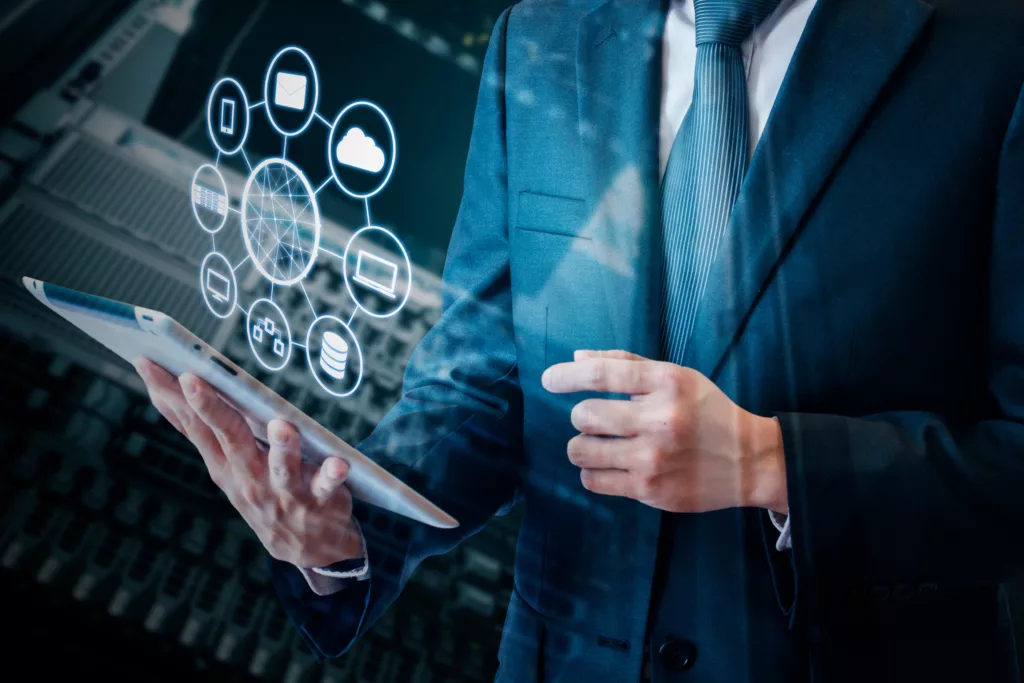
The Best Visualization Tool for Wonderware Historian
Unlocking the full potential of your Wonderware Historian requires powerful visualization and analytics tools that ...
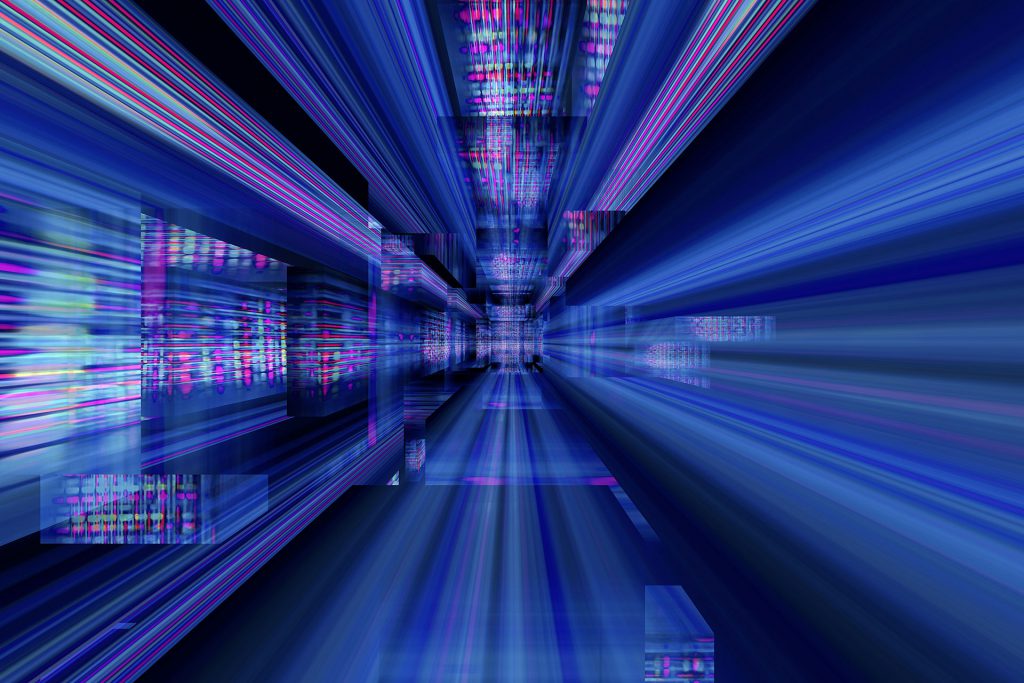
dataPARC Introduces AWS and Azure-Compatible Data Historian Platform for Advanced Industrial Applications and Cloud AI/ML Integration
Latest data historian release is optimized to share OT data for real-time plant operations and ...
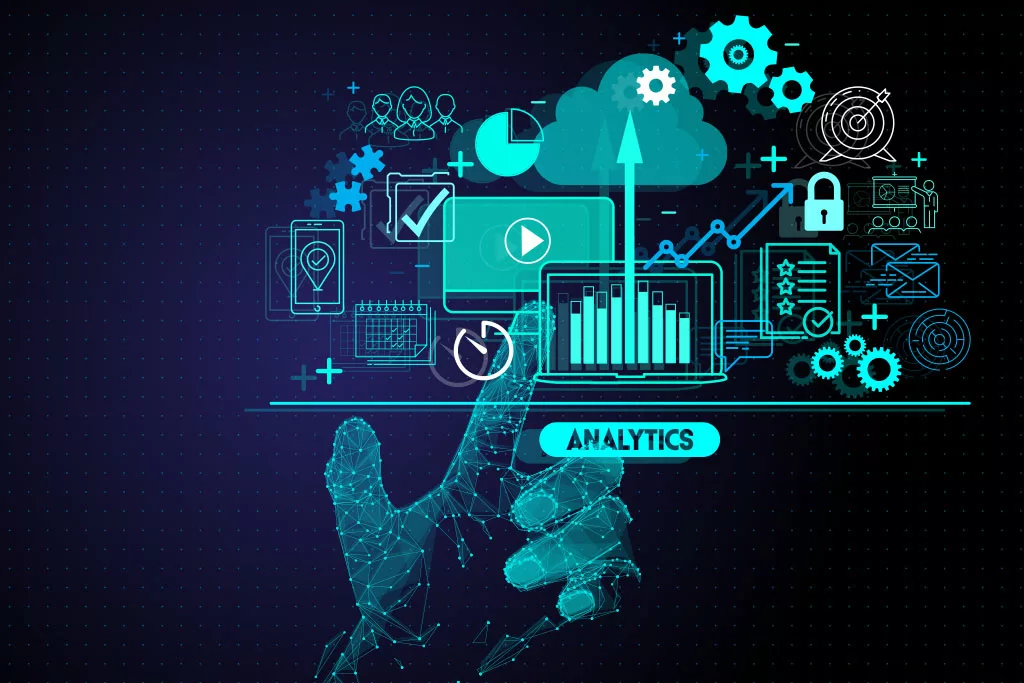
Breaking Bottlenecks: Utilizing Maximum Sustainable Rate Analysis in Manufacturing
In this blog post, we’ll dive into what Maximum Sustainable Rate (MSR) analysis is, why ...
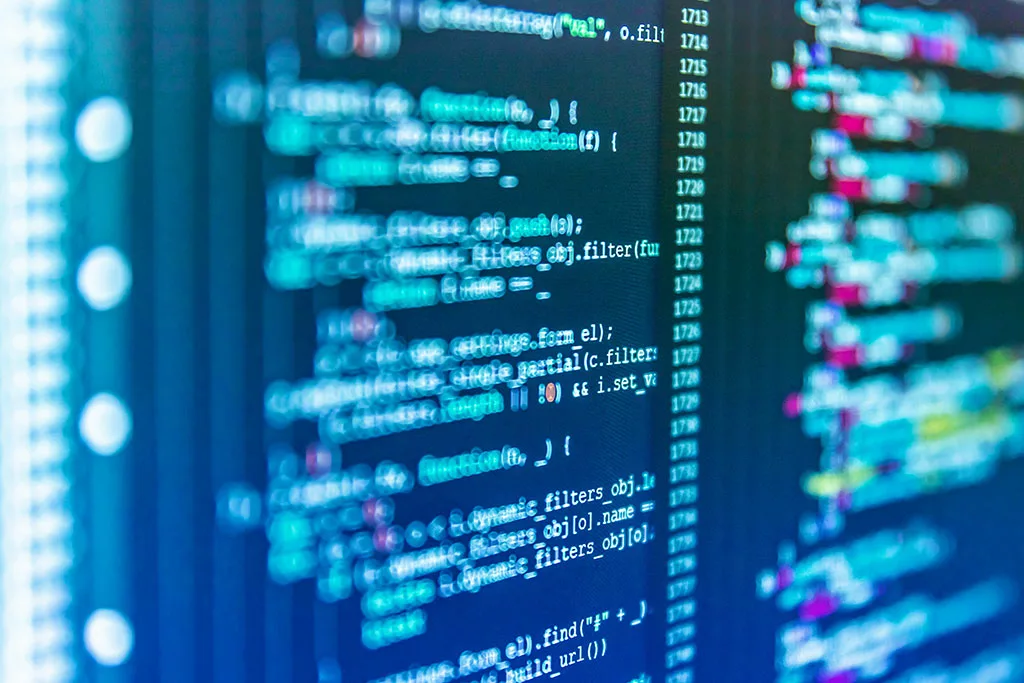
Best Time-Series Databases for Third-Party Applications
In this article, we will compare several popular time series databases and evaluate their suitability ...
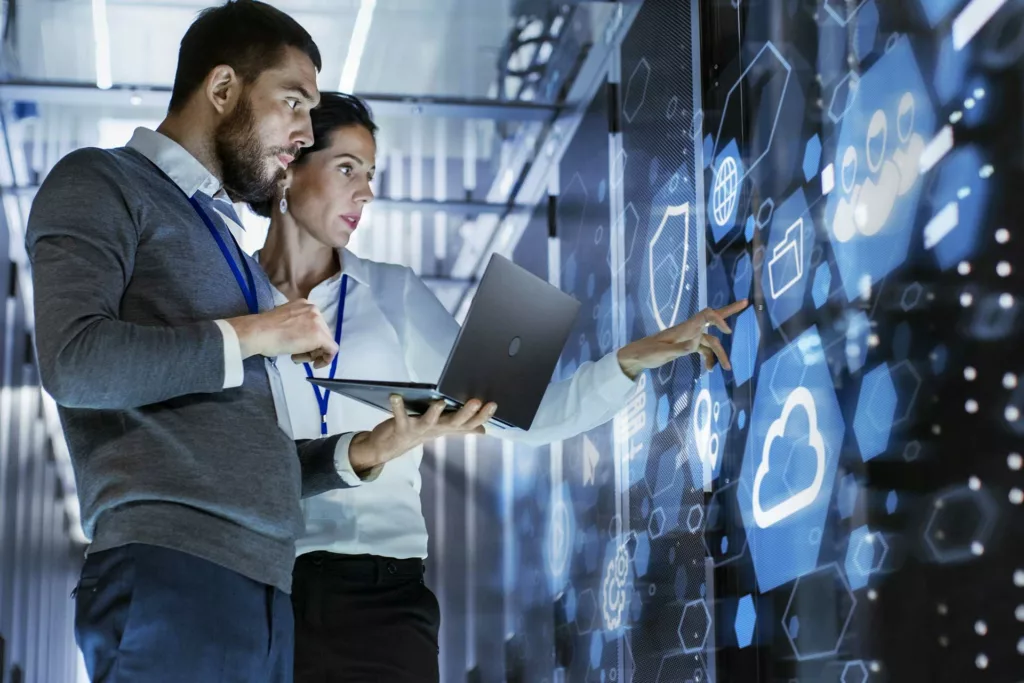
What to look for in a Data Historian for Third-Party Integration
Not all historians are created equal. Throughout this article, we will explore what to look ...
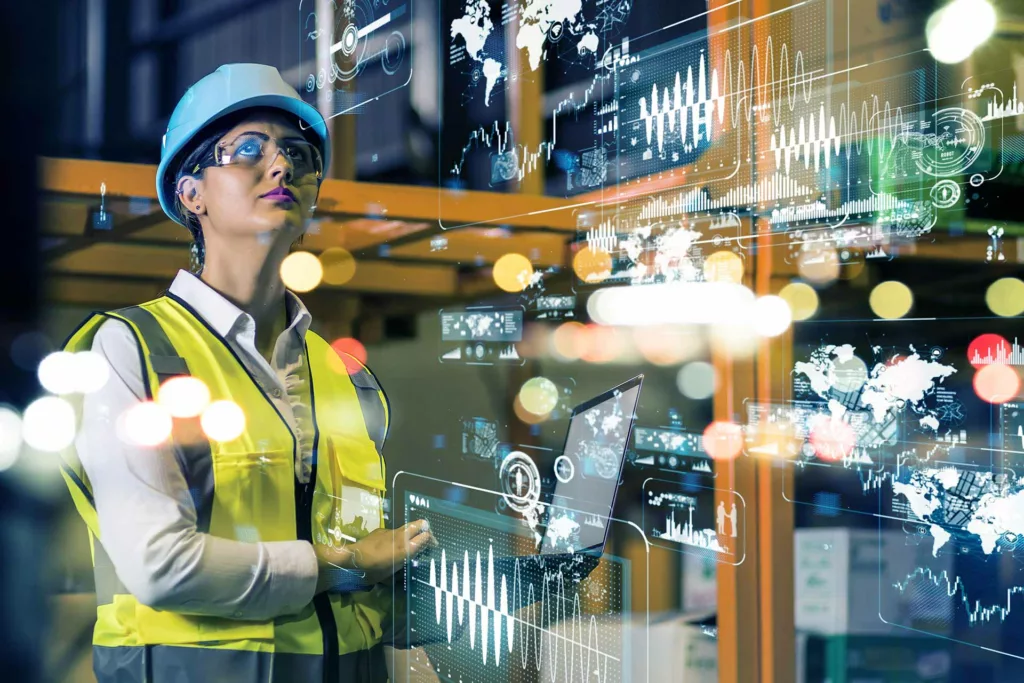
The Future of Manufacturing: Digital Transformation
Digital transformation is changing the manufacturing industry. It enables companies to increase productivity, make data-driven ...
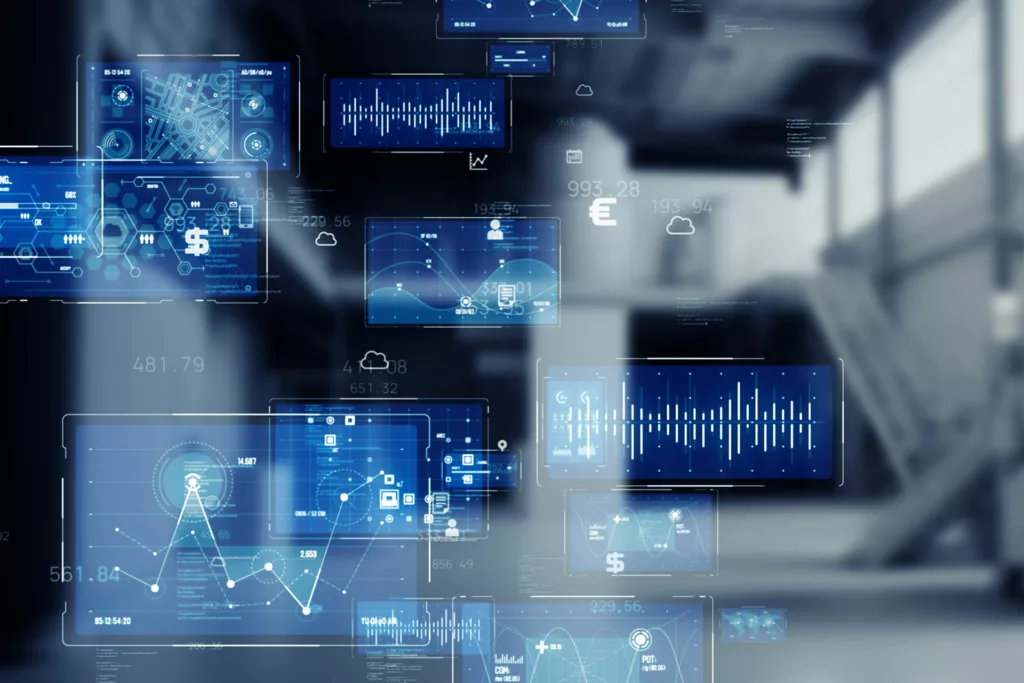
Advanced Analytics vs. Operational Analytics in Manufacturing
The interest in and application of industrial analytics has grown rapidly in recent years, driven ...
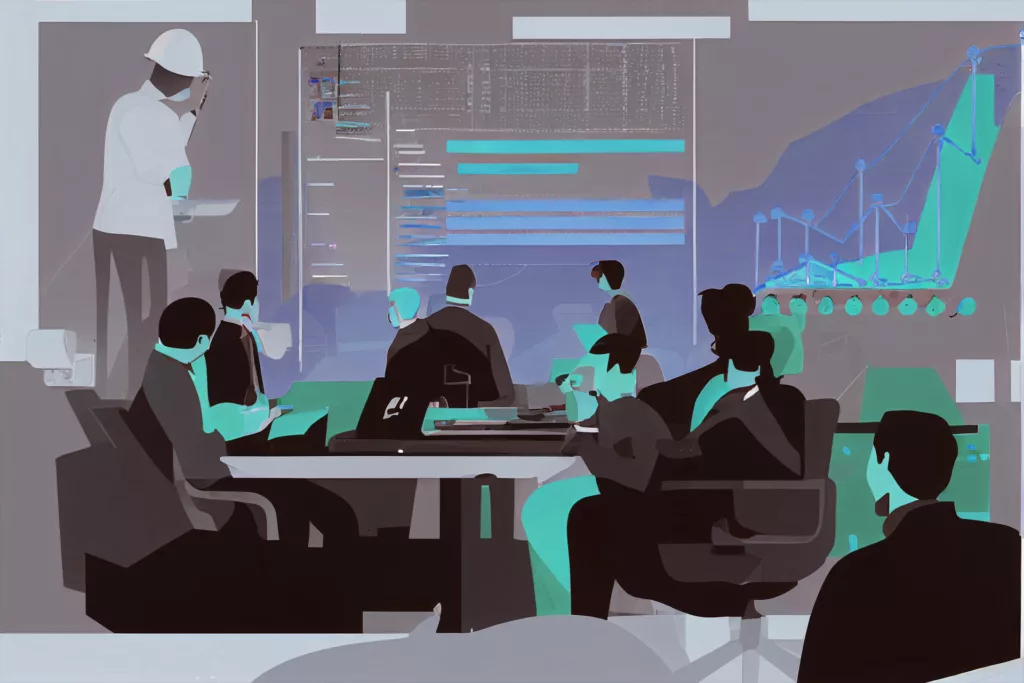
Mastering Operational Reviews: a Guide to DORs, WORs, & MORs
Reviewing operational data and KPIs are important for daily operation and communication up to management. ...
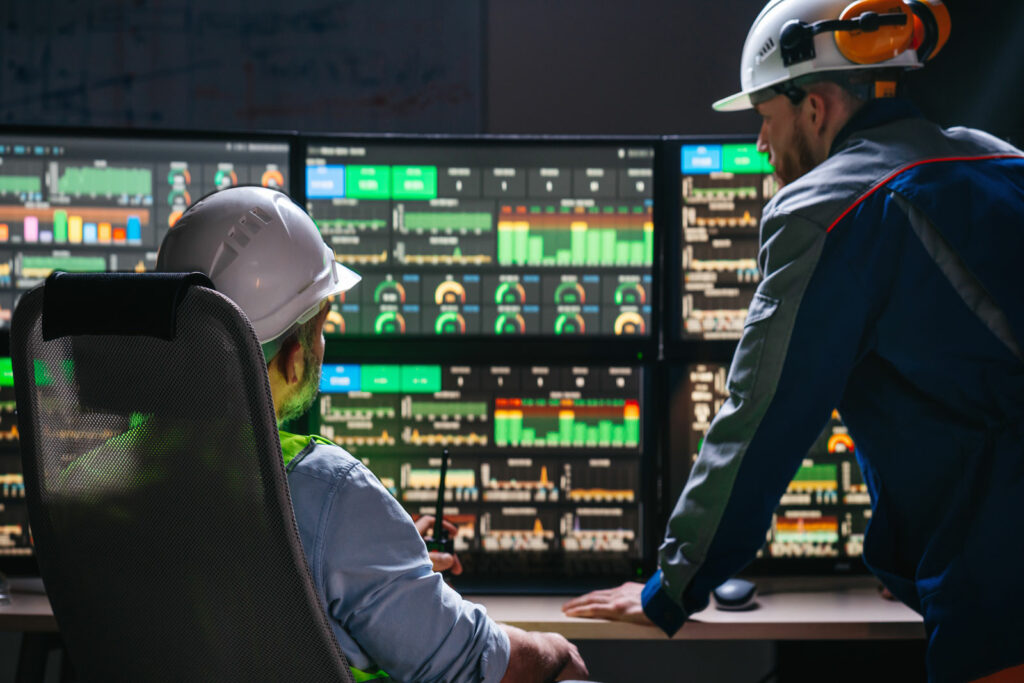
Building Effective Manufacturing KPI Dashboards and Reports
Graphical dashboards are a great way to display information to a wide audience. By building ...