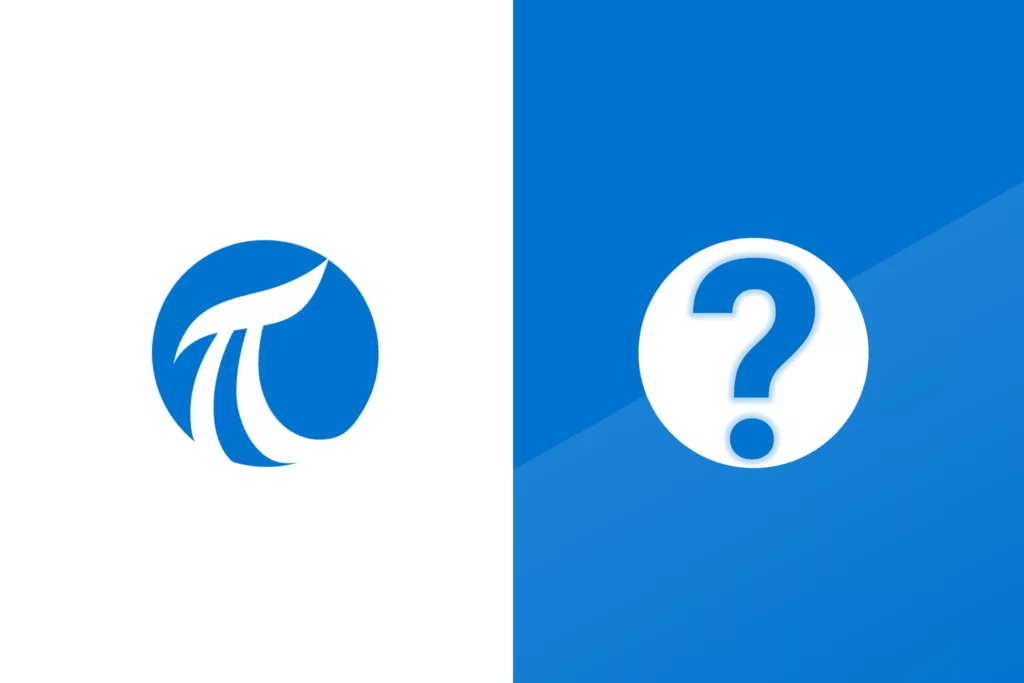
Best Pi Vision Alternatives [2025]
When it comes to process data visualization and analysis, PI Vision is a top choice ...
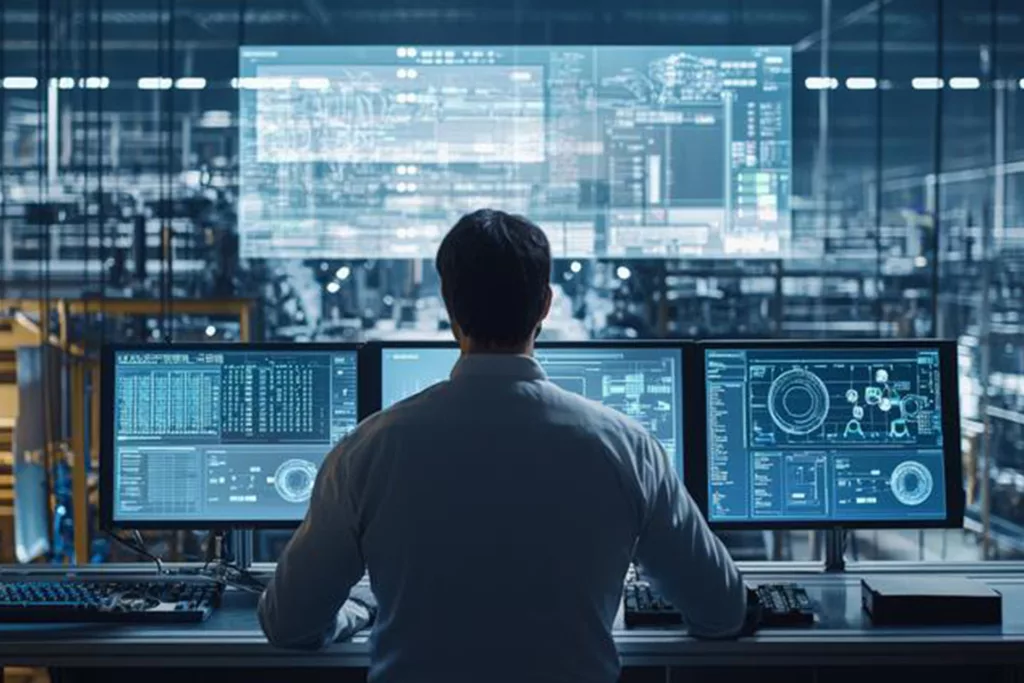
3 OEE Dashboard Templates for Smarter Manufacturing
In this blog, we’ll introduce a set of downloadable OEE dashboard templates designed for manufacturing ...
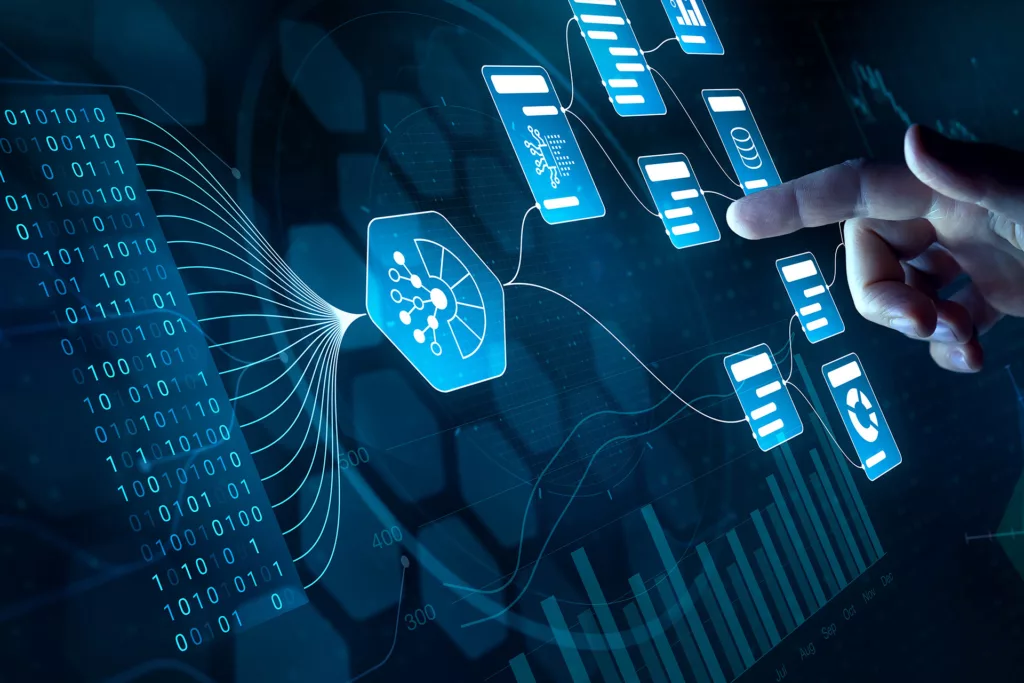
Unified Namespace Software: Tools Manufacturers Use to Connect IT & OT
In this blog, we’ll take a closer look at unified namespace software and why it’s ...
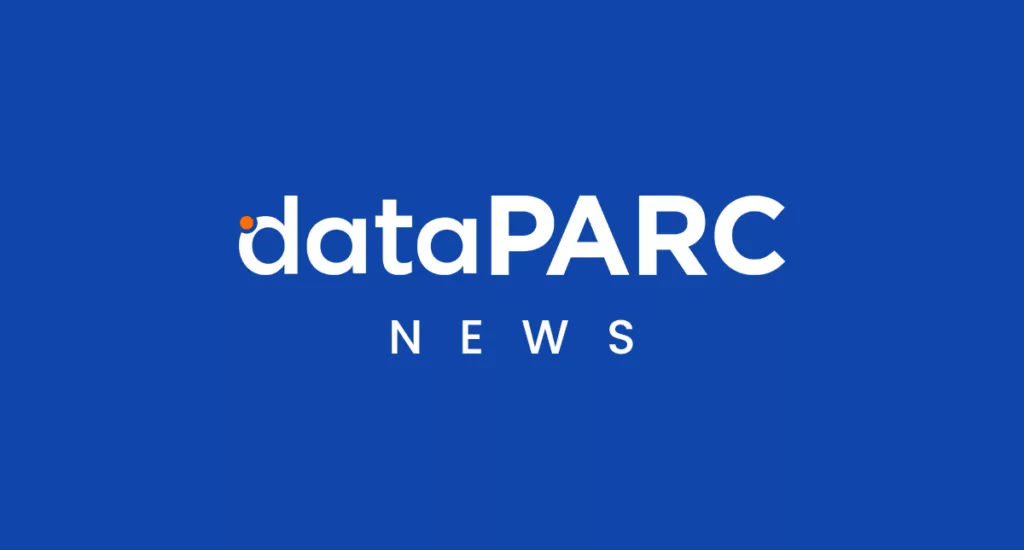
dataPARC Recognized as a Top Work Place 2025
dataPARC has been recognized as a top workplace in Engineering.com’s workplace survey, earning a 93% ...
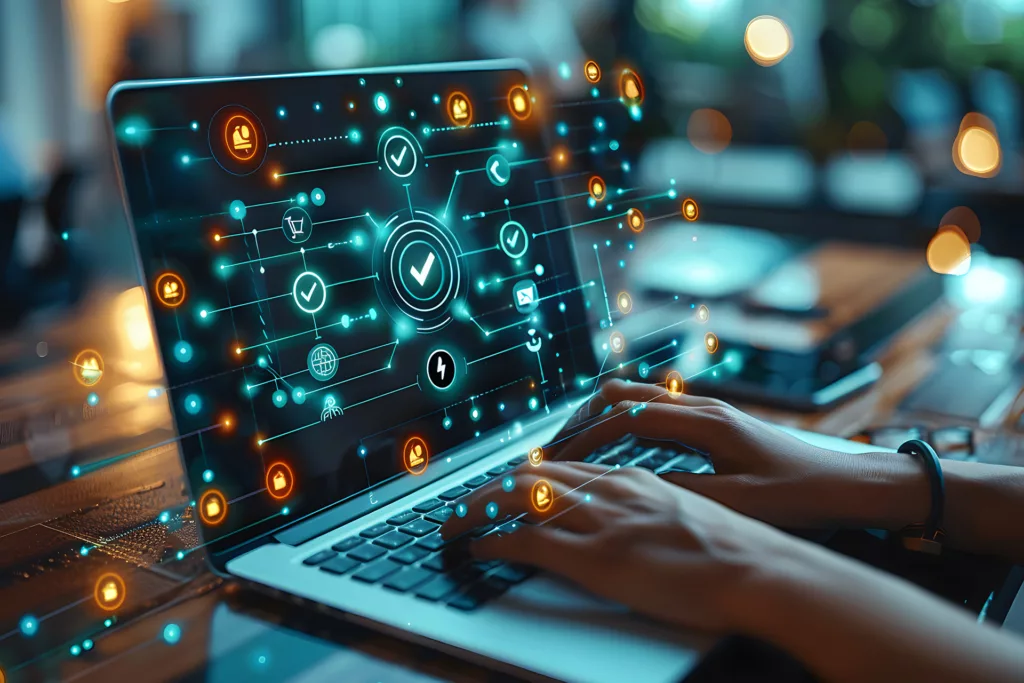
Unified Namespace (UNS) Explained: A Key to Industrial Data Visibility
In this blog, we’ll explain a Unified Namespace (UNS), how it streamlines industrial data management, ...
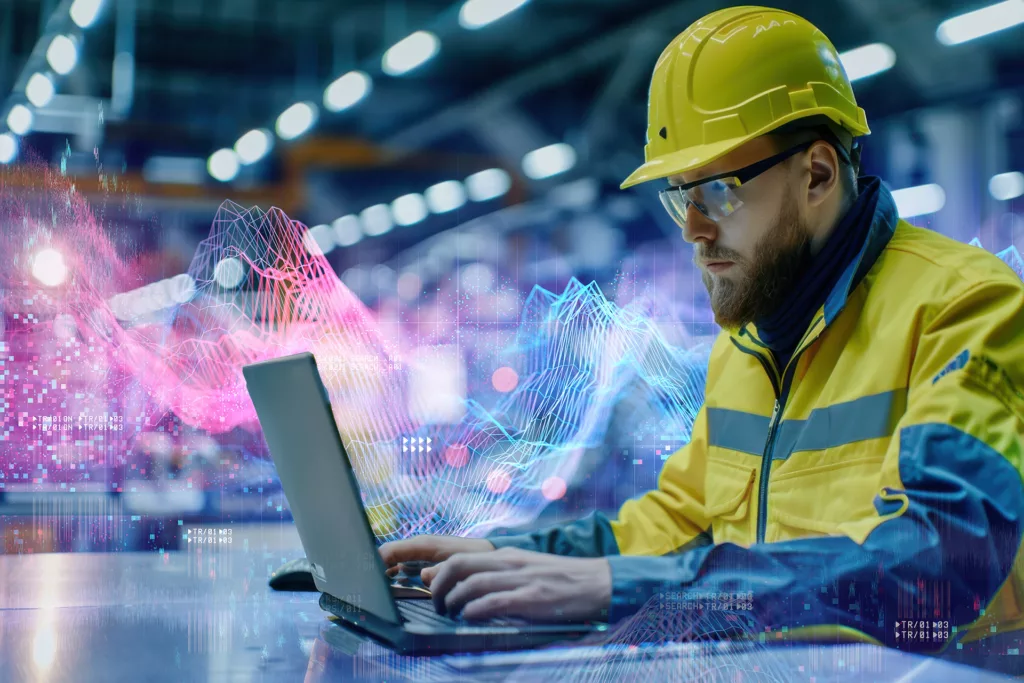
Yokogawa Exaquantum Alternative for Effective Data Management
Discover an effective alternative to Yokogawa Exaquantum for streamlined data management. Find the best solutions ...
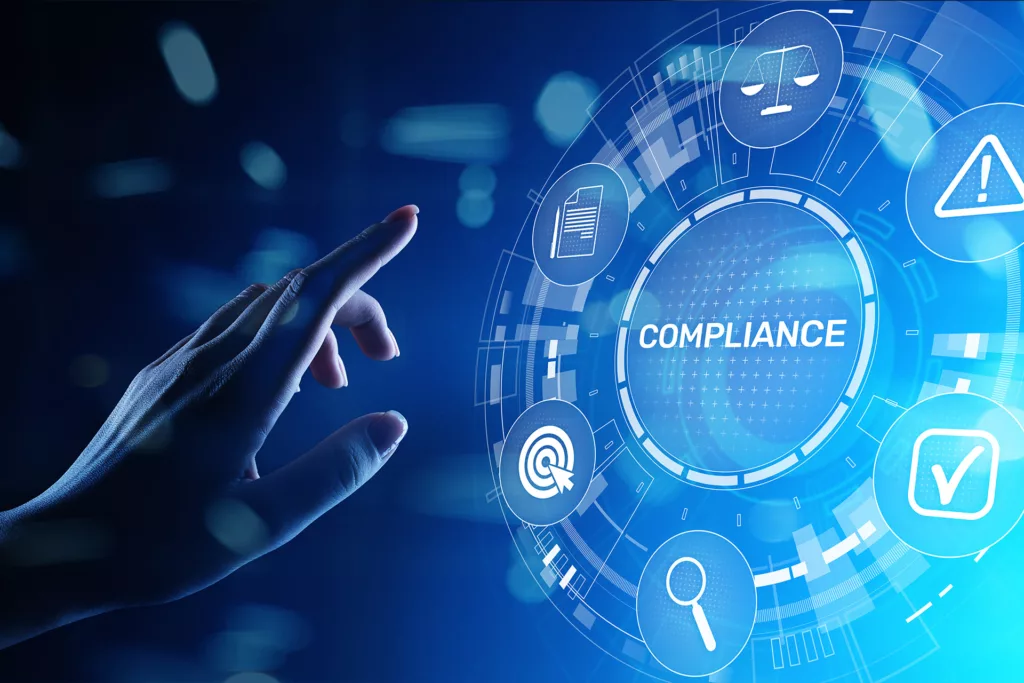
Ensuring 21 CFR Part 11 Compliance with dataPARC Suite and Historian
21 CFR Part 11 compliance is essential for those in regulated industries. In this article ...
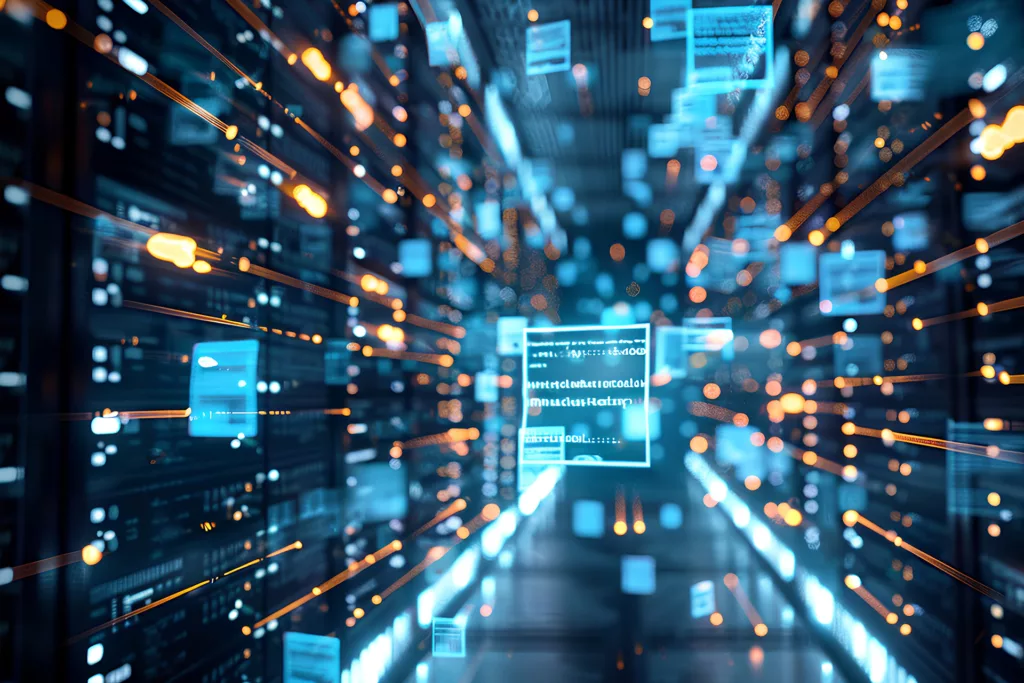
From Data Silos to AI: Ready Your Manufacturing Ecosystem for AI
In this article, we will explore the necessary foundational elements of a data ecosystem for ...
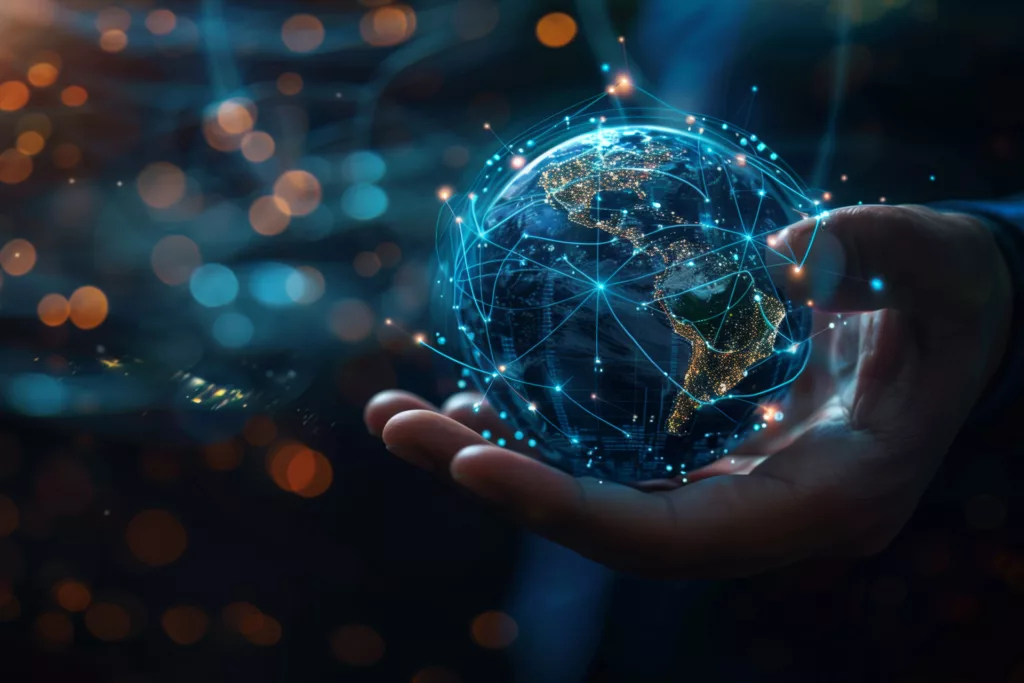
Upgrade Your Industrial Software: Best GE Proficy Replacement
This article explores the key considerations for a GE Proficy replacement. We’ll discuss the benefits ...
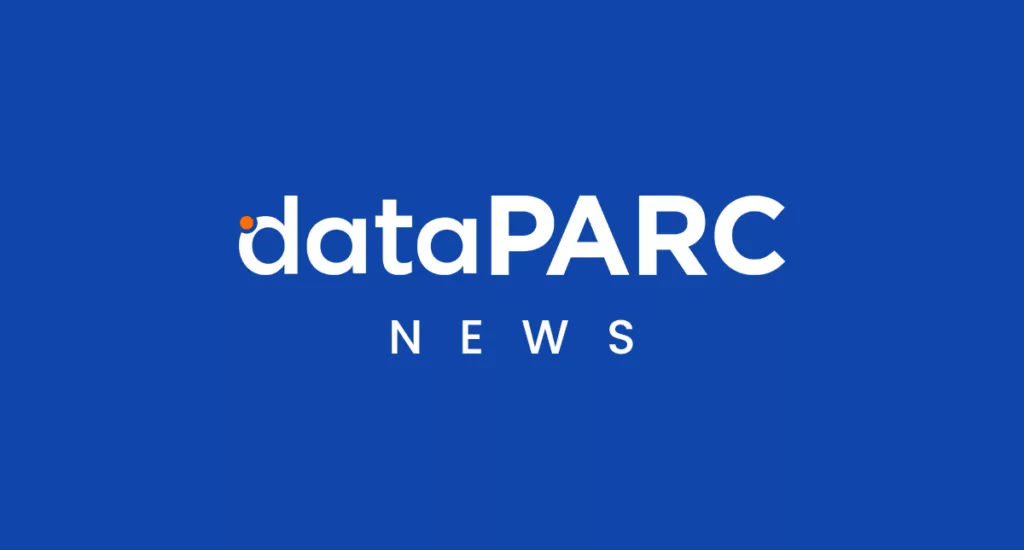
dataPARC Unveils Browser-Based Version of PARCview, Extending Process Data Visualization and Analysis Across Devices
New web application complements the powerful desktop client, offering real-time monitoring and analysis on any ...
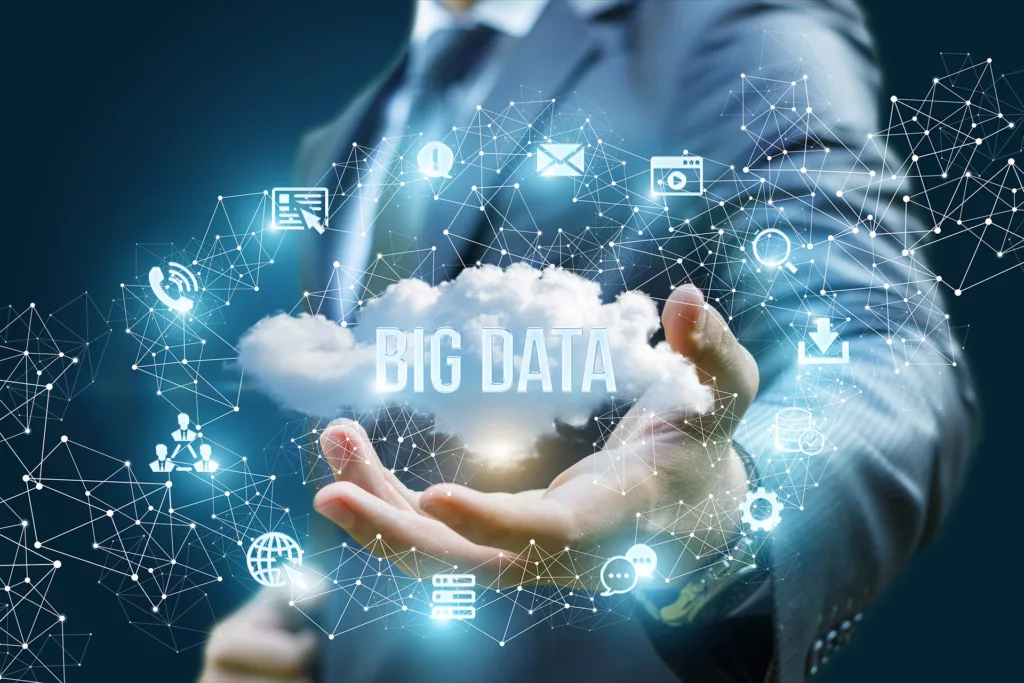
The Role and Importance of Big Data in Manufacturing
Big data is reshaping the manufacturing landscape by unlocking new levels of efficiency, quality, and ...
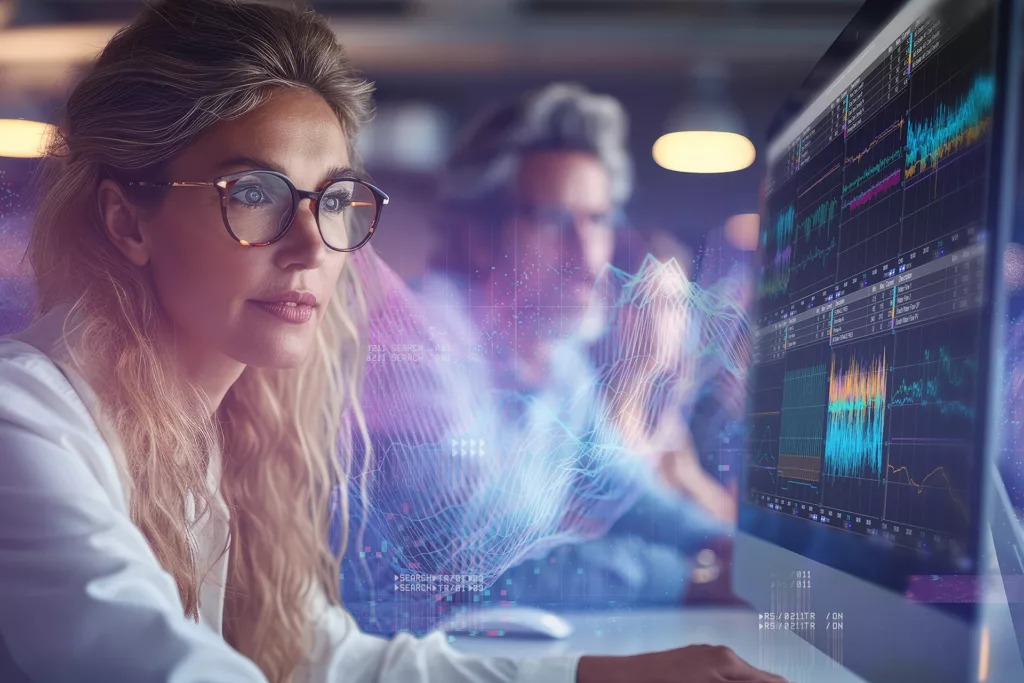
Boosting Workflow Efficiency: What to Add to Your Data Management Scorecard
In this article, we’ll explore the essential elements of a Data Management Scorecard and introduce ...
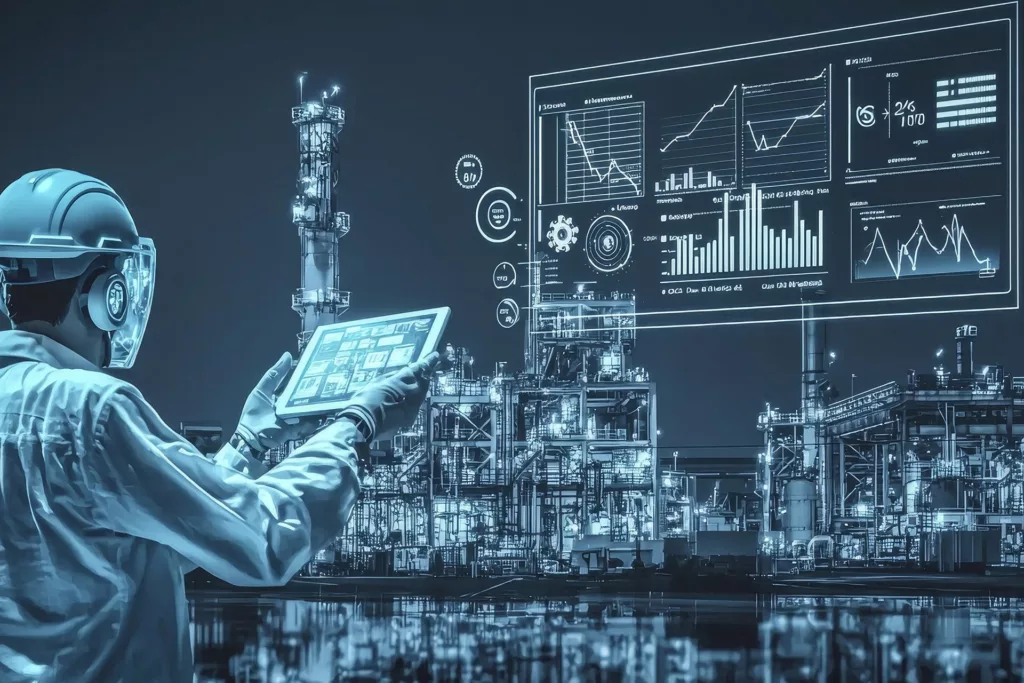
Understanding Digital Twin Platforms: Transforming Data into Actionable Insights
Digital twin platforms are revolutionizing how manufacturers approach data, enabling real-time monitoring, predictive maintenance, and ...
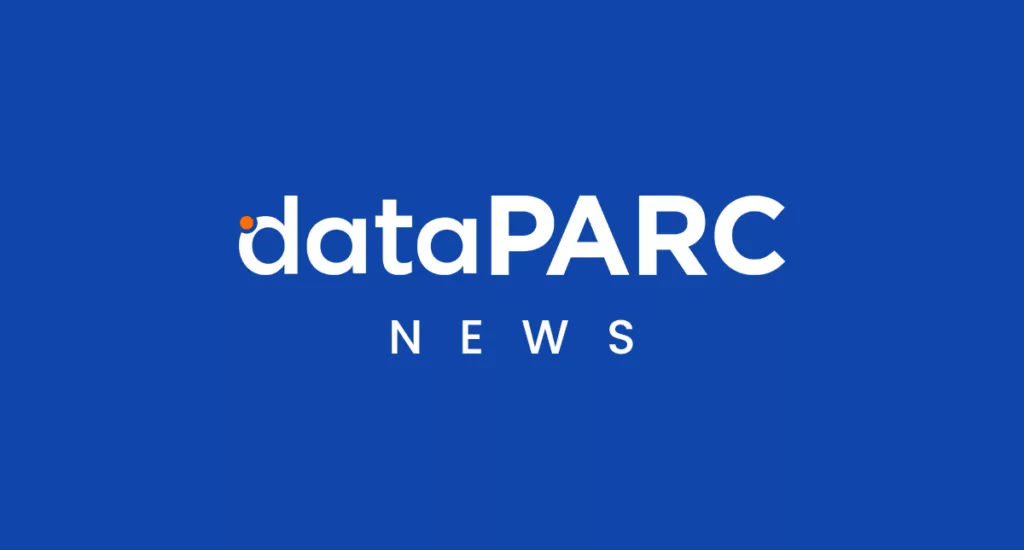
Georgia-Pacific Selects dataPARC for Process Data Visualization and Analysis Across Containerboard and Cellulose Divisions
Washougal, WA – July 31, 2024 – Georgia-Pacific, a global leader in tissue, pulp, paper, ...
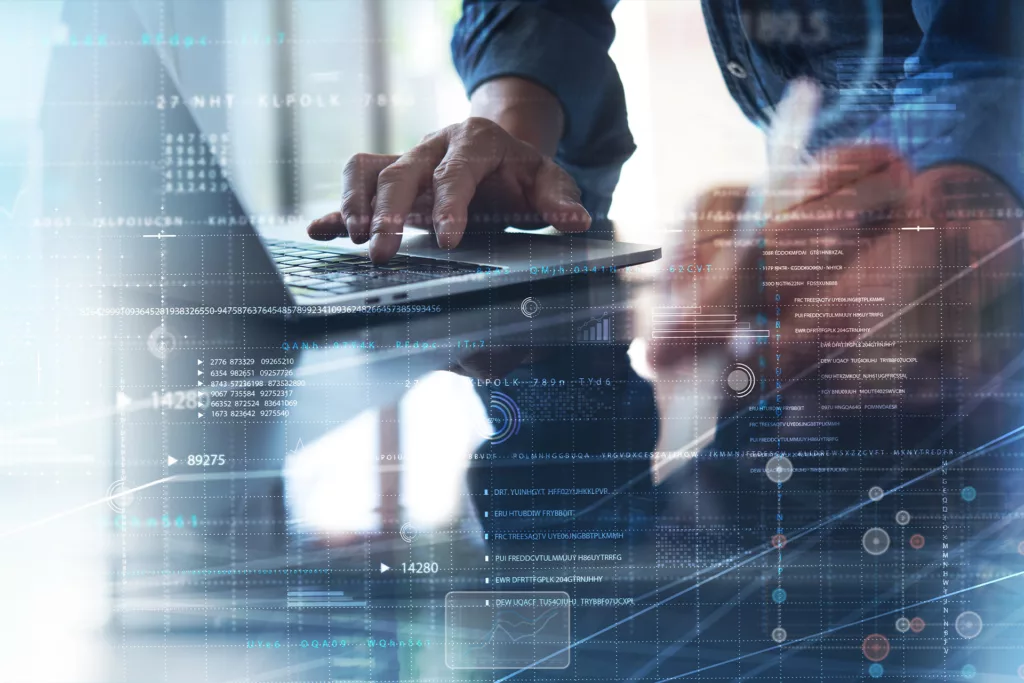
3 Top PI System Alternatives to Collect and Visualize Data
With PI ProcessBook being phased out, many companies are looking into PI System Alternatives. Managing ...
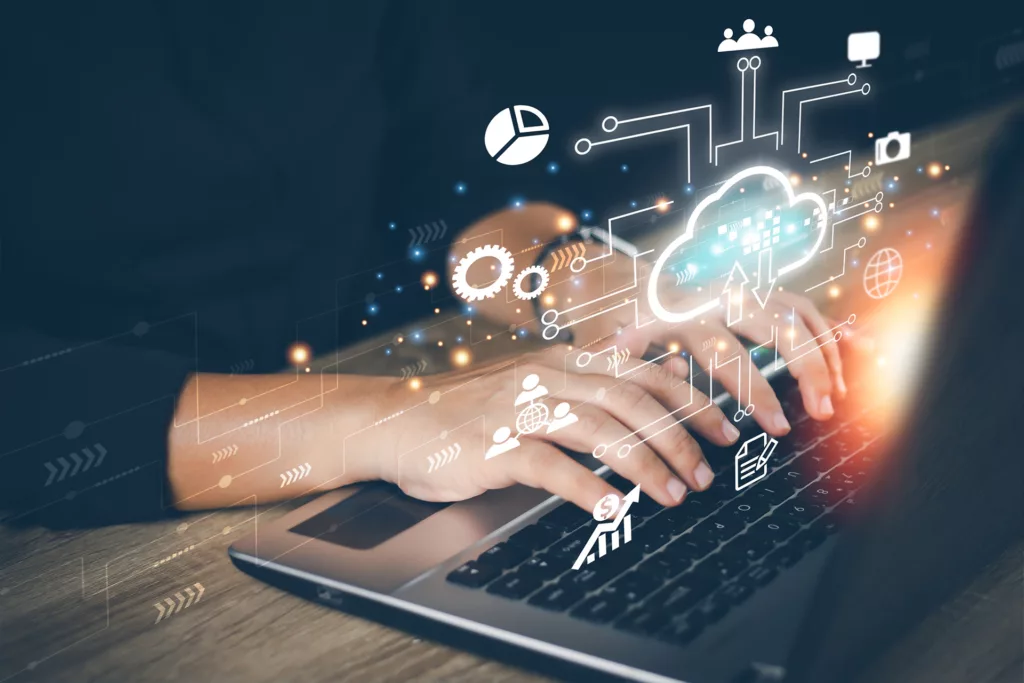
Importance of an Industrial Data Platform for Manufacturing Data
In this blog, discover how Industrial Data Platforms can provide increased productivity, lowered costs, and ...
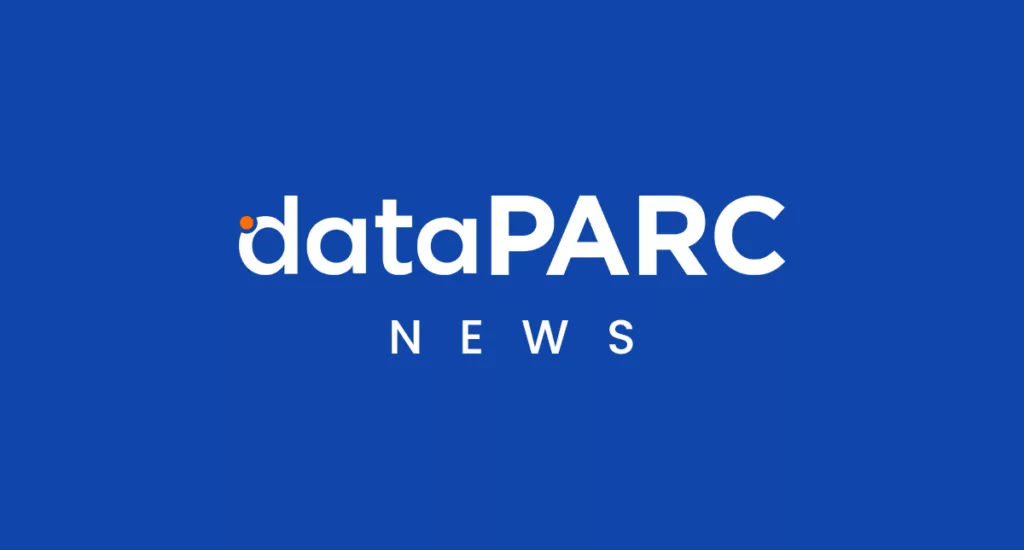
dataPARC Expands Operations in Latin America to Meet Growing Demand
Washougal, WA – dataPARC, a leading provider of industrial analytics and process optimization solutions, announced ...
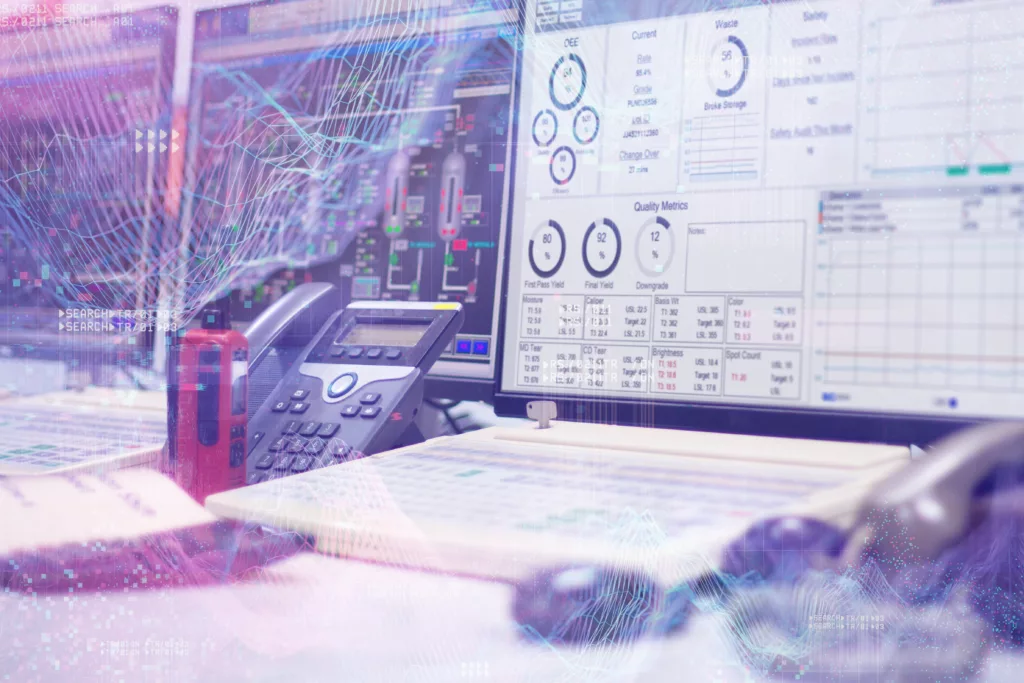
OEE Data Collection Software: Reducing Waste and Increasing Efficiency
In this blog, discover how OEE data collection software can significantly boost efficiency, minimize waste, ...
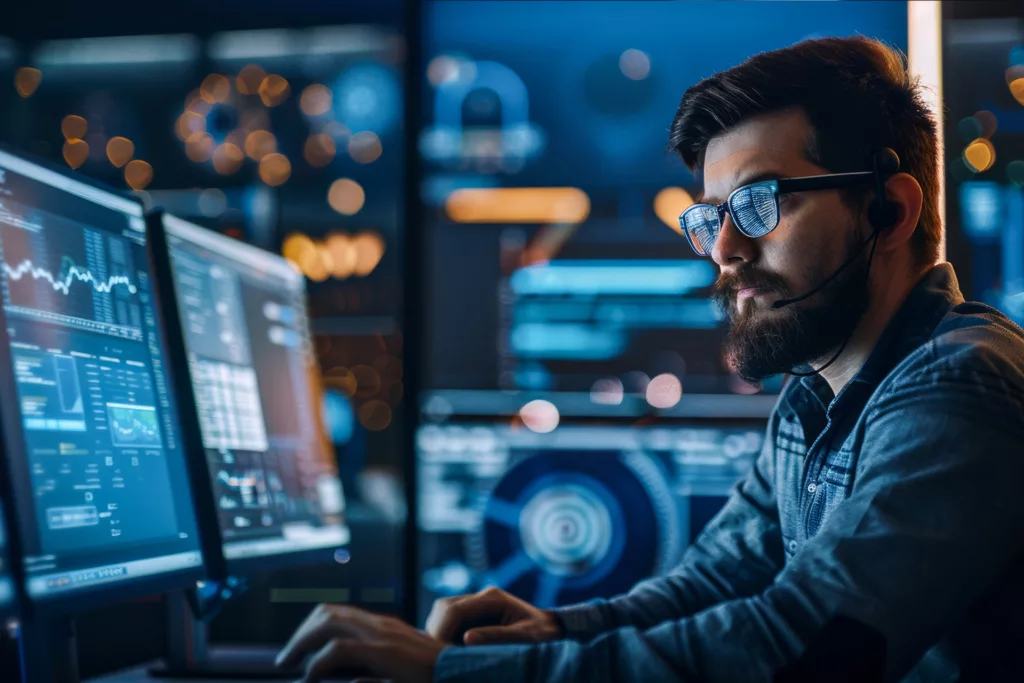
Real-Time Manufacturing Dashboards: Setup, Importance, and Benefits
In this blog, explore what real-time manufacturing dashboards are, why they are crucial, and how ...
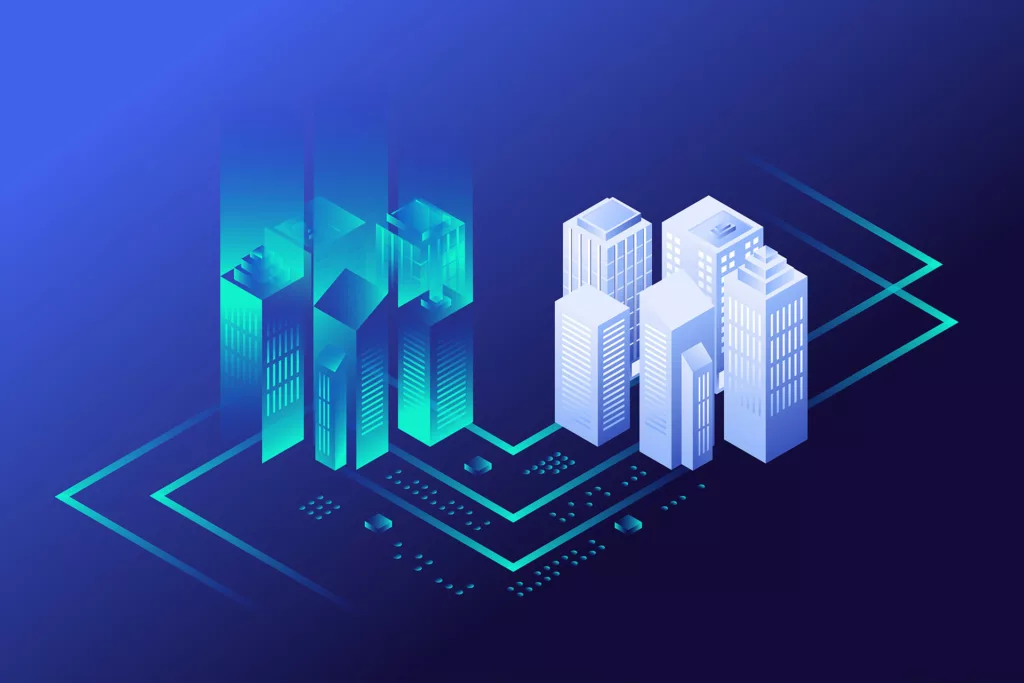
Digital Twins in Manufacturing Explained: Key Advantages and Implementation Insights
Uncover how a digital twin in manufacturing can revolutionize your production processes. Learn how digital ...
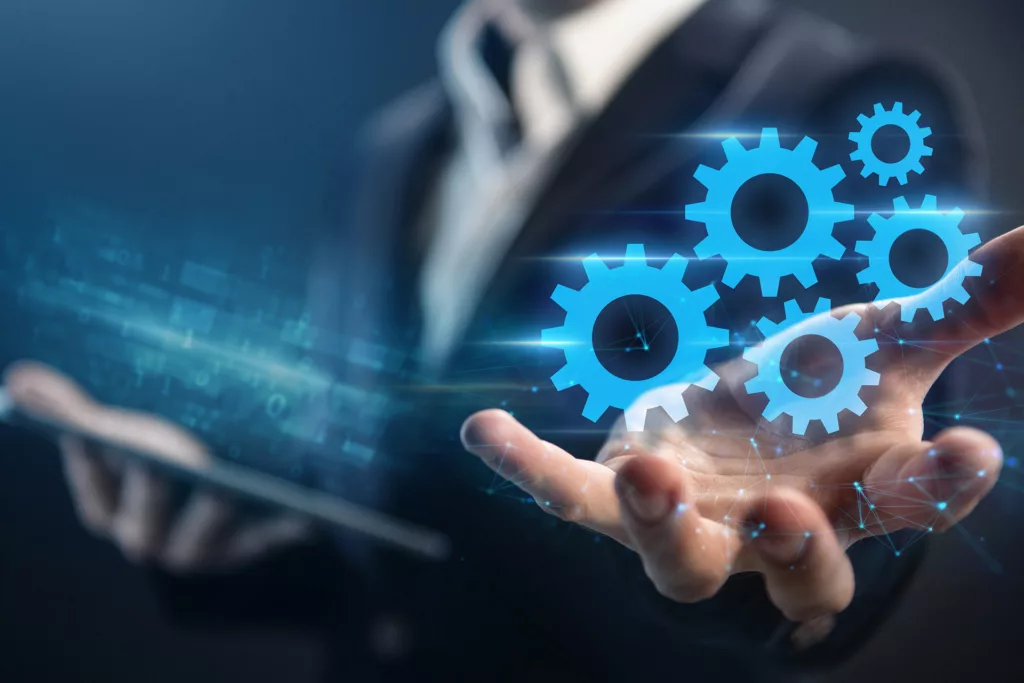
Manufacturing Process Optimization Strategies
Learn about manufacturing process optimization strategies that can boost your output when paired with the ...
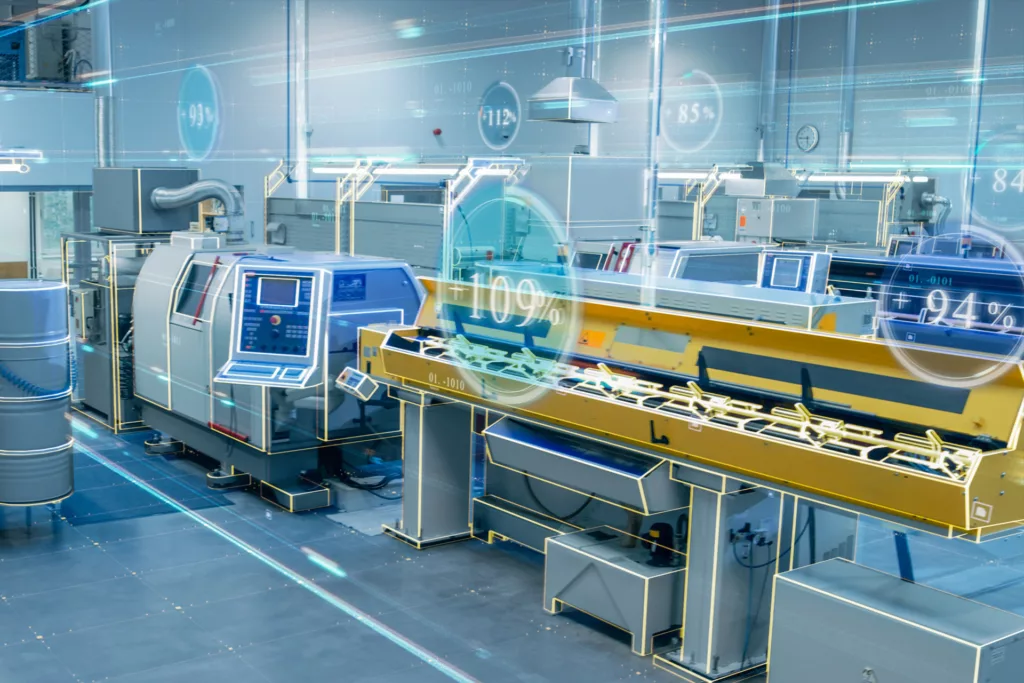
How to Improve Manufacturing Efficiency in 4 Steps
This blog explores four strategic steps to improve manufacturing efficiency: engaging workers, harnessing data intelligence, ...
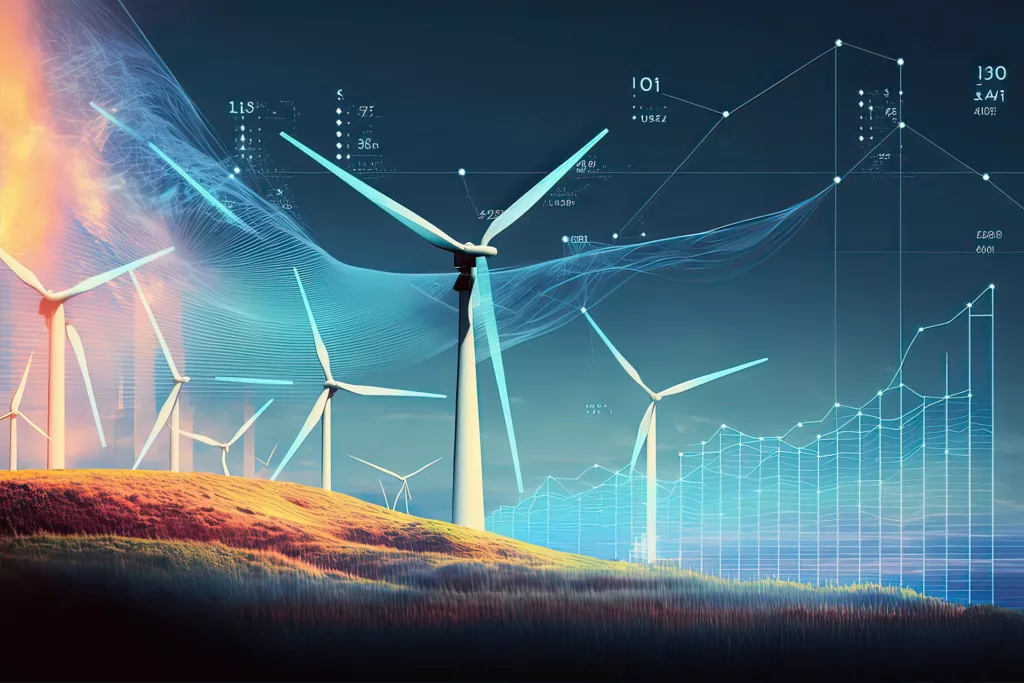
Industrial Energy Management: Track Usage and Gain Efficiency
Learn how to manage energy at your site, an increasingly vital strategy for industries aiming ...
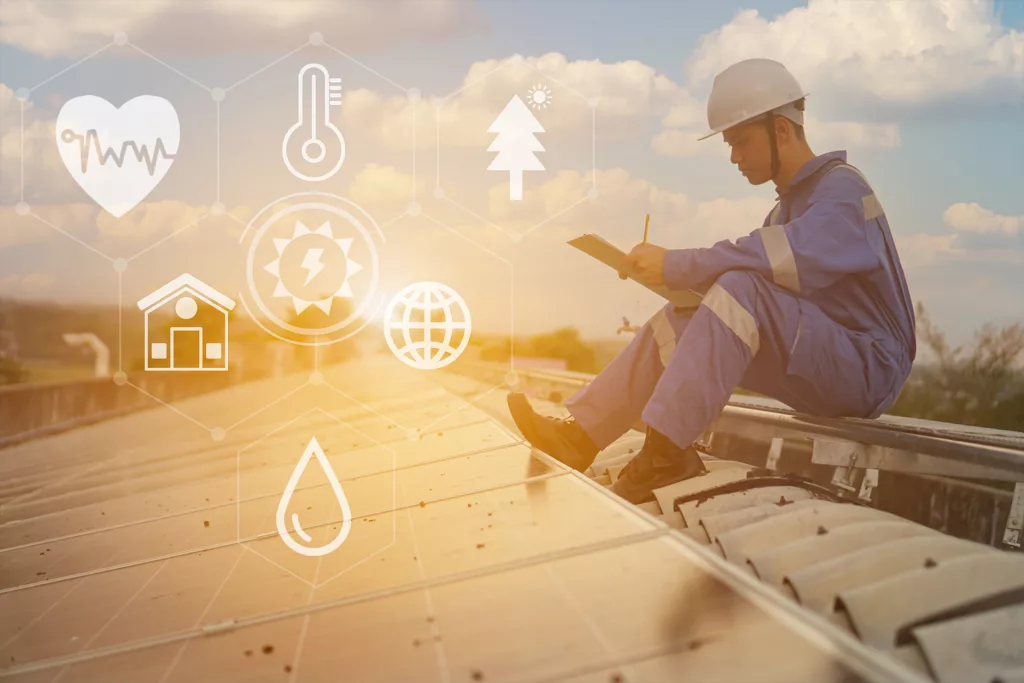
What is Industrial Environmental Monitoring and How to Do It
This blog explores the essential aspects of Industrial Environmental Monitoring, emphasizing its role in promoting ...
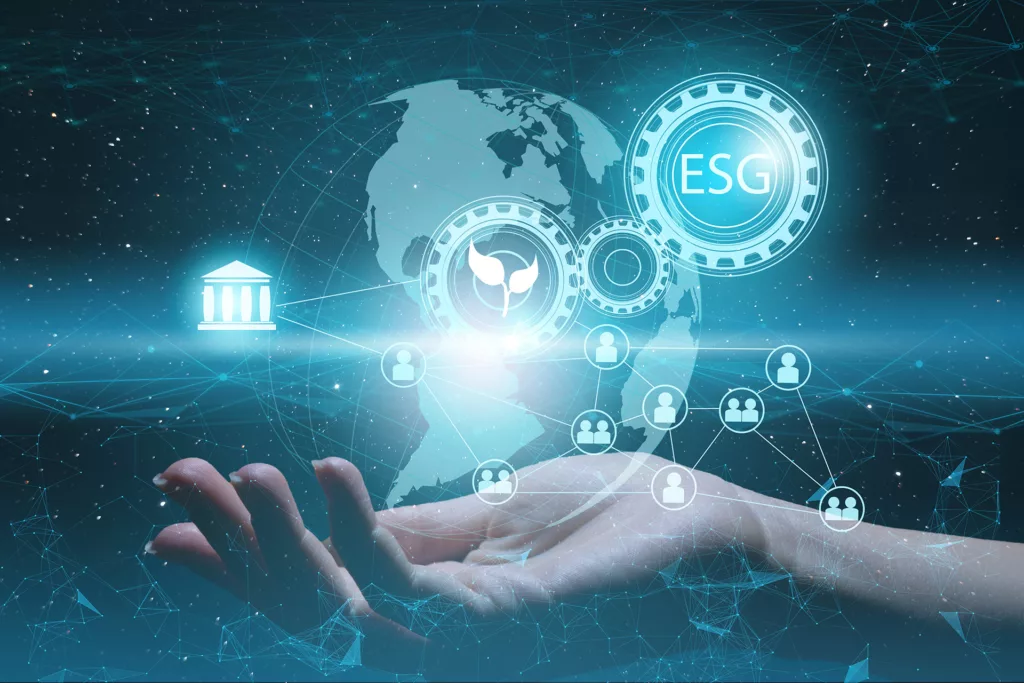
Optimizing ESG Compliance with Environmental Data Software
As businesses adjust to environmental data demands in ESG compliance, understanding the needs of environmental ...
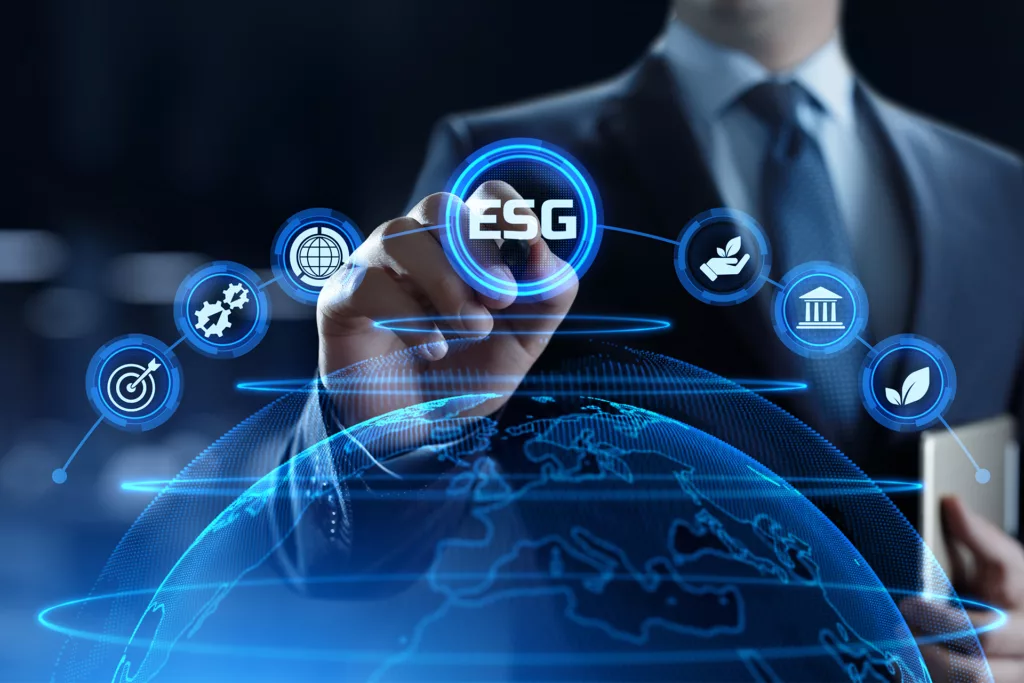
ESG Data Analytics is a Game-Changer for Manufacturing
In this blog post, we will explore ESG data analytics, how it pertains to manufacturing, ...
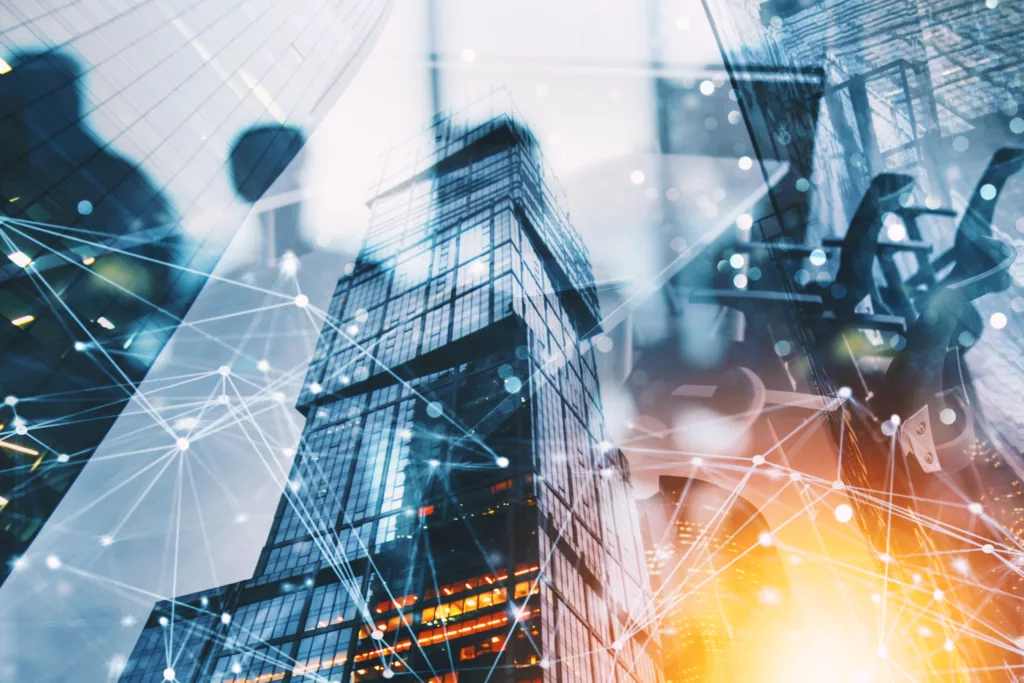
Discussing FactoryTalk VantagePoint Replacements
As the impending discontinuation date of Rockwell Automation’s VantagePoint draws near, manufacturers find themselves presented ...
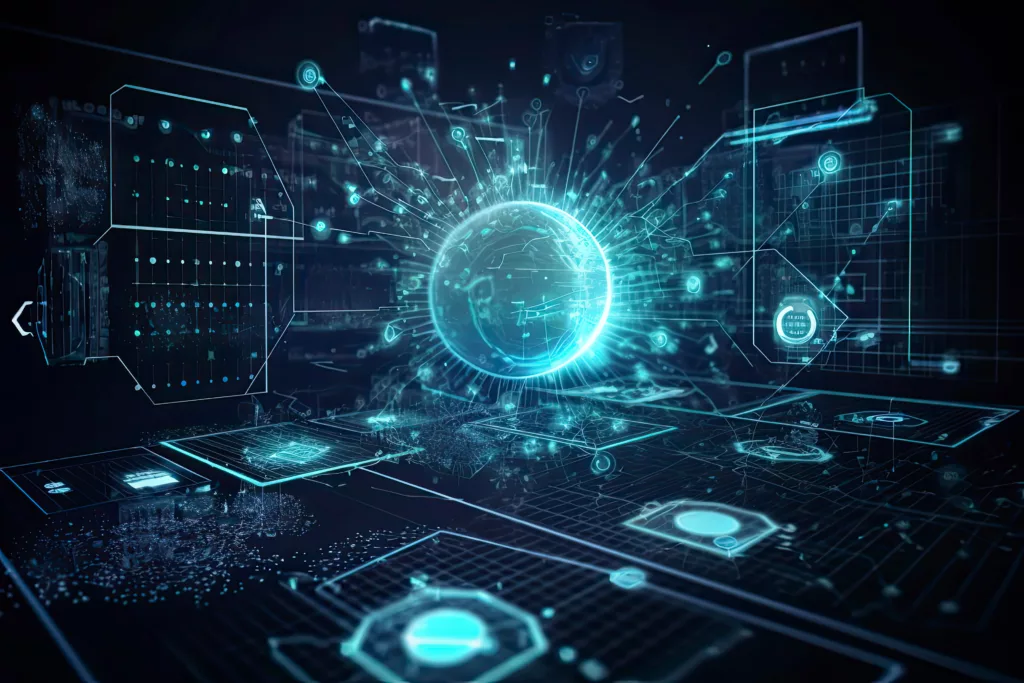
dataPARC Wins 2023 Breakthrough Product Award for its Cutting Edge Data Historian
Nashville, TN – dataPARC has been honored with the prestigious 2023 Breakthrough Product Award, as ...