In this article, we will explore the necessary foundational elements of a data ecosystem for AI needs in manufacturing. We will discuss how it takes a well-managed data ecosystem for AI development. Big data and a strong foundation create new opportunities for efficiency, optimization, and strategic growth. By understanding the steps required to prepare and strengthen your data ecosystem, manufacturers can unlock the full, transformative potential of AI and seamlessly integrate it into their business processes.
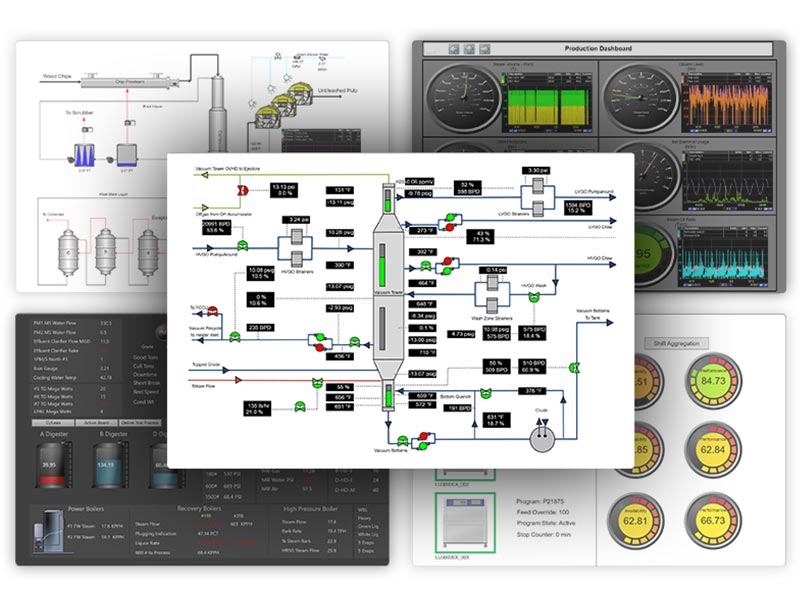
Ready your Manufacturing Ecosystem with a Fast Data Historian and Process Monitoring
Why Do Data Ecosystems Matter?
For competitive manufacturers, a strong data ecosystem is critical for successfully implementing advanced technologies like Artificial Intelligence (AI) and Machine Learning (ML). There are numerous examples of companies attempting to implement complex AI and ML strategies prematurely. This has led to costly failures. When a data ecosystem isn’t mature enough, moving forward with highly advanced technologies can result in major setbacks for businesses, both financially and operationally.
The challenges of an underprepared data ecosystem go beyond just monetary losses. Implementing AI and ML without a strong foundation can erode trust among employees by not having consistent or complete data. This can lead to a lack of confidence in the system and, ultimately, poor user adoption. Additionally, IT/OT teams can become overwhelmed with the increased workload of troubleshooting, patching, and managing data inconsistencies, further straining resources.
A well-prepared data ecosystem, on the other hand, sets the stage for smoother artificial intelligence integration. This involves having a reliable data infrastructure, robust data pipelines, governance frameworks, and tools for data analysis that facilitate collaboration across teams. It is important to prepare your site adequately. A site with clean, accessible, and well-integrated data means that AI-driven solutions can be leveraged effectively. This leads to more insightful analysis and proactive decision-making.
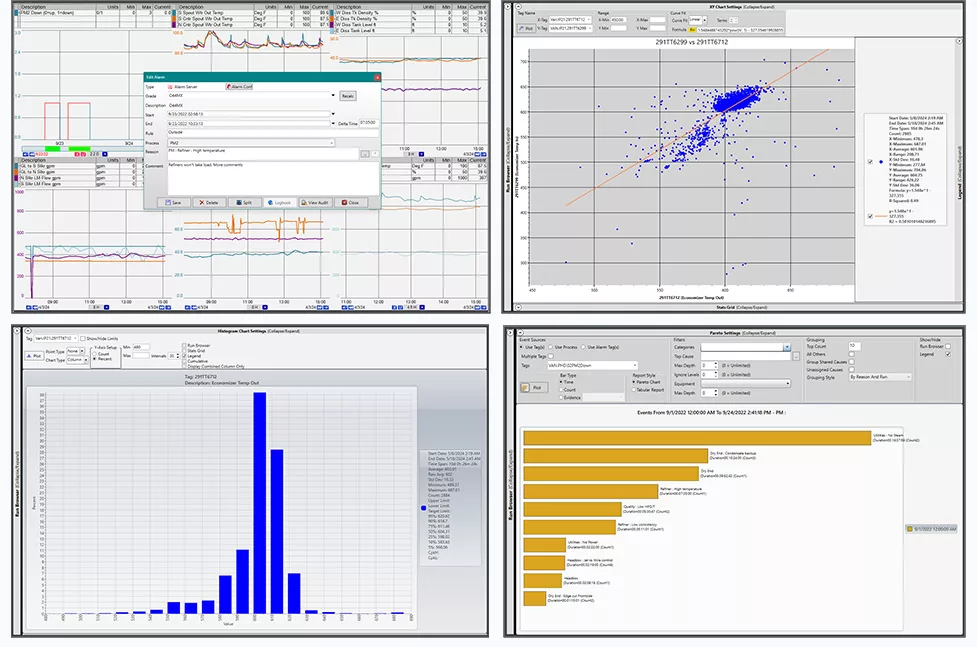
Access all critical data from a single platform to be able to visualize and analyze it for continuous improvement. From lab systems to process data, see it all in one place.
Manufacturers need to assess their current data ecosystems to identify gaps and areas for improvement before diving into artificial intelligence. This preparation is not solely focused on the technology itself. It includes fostering a culture that embraces data-driven decision-making and encourages continuous data utilization and integration. By doing so, your operations can move beyond basic process automation. You can harness the full potential of AI and ML to drive optimization and innovation.
Evaluating My Data Ecosystem for AI
First, you have to evaluate the current state of your manufacturing data ecosystem to determine its readiness for AI and ML initiatives. One effective method is using the digital transformation roadmap, which outlines five levels of data maturity. A site should be at level 4 or higher to implement artificial intelligence successfully. Let’s review each stage, highlighting the progression from basic data availability to a robust data-driven culture.
Stage 1 – No Data, No Time
At Stage 1, manufacturing and operations decisions are largely based on intuition or longstanding practices, with minimal reliance on data. Any available data is often raw, manually collected, and typically stored as paper records or basic spreadsheets.
This stage lacks formal data storage solutions. There is a lack of structured data management, meaning data quality is inconsistent. The data ecosystem for AI here is practically non-existent, severely limiting value creation and efficiency improvements. It would be unreasonable to attempt to implement any AI or ML integrations at this stage.
Stage 2 – Some Data, Still Little Time
In Stage 2, there is some level of automation begining to supplement manual data collection. However, significant gaps still remain. Data is flowing in limited amounts. That data is often only accessible by specific departments and may be stored in data silos.
The site might have rudimentary data storage mechanisms. Without a centralized data historian, data is not accessible. Decisions are still largely reactive and based on raw data with questionable quality. This stage is where data initiatives start but may not yet provide the comprehensive, trustworthy insights needed for broader use. The data ecosystem for AI still has a ways to go.
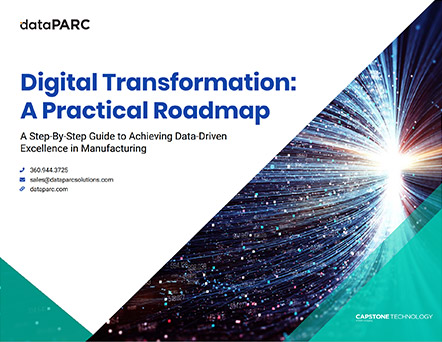
Working on digital transformation at your plant? Let our Digital Transformation Roadmap guide your way.
Stage 3 – Reflective Decision-Making
By Stage 3, data has become part of the decision-making process. It is supported by the implementation of a data historian. This step improves the reliability of data storage and offers a more consistent view of raw data across departments. However, data silos still exist, preventing seamless access to a single source of truth.
Data quality at this stage begins to improve. This allows for more meaningful analysis, yet integration across various stakeholders in the data ecosystem is limited. The site can start basic data analysis. Which facilitates reflective decision-making that leverages data to review past performance but not yet predictive insights. Many manufactuers are at this stage as they try to implement AI and ML tools. Although data has started making its way throughout the organization, foundational aspects still need to be addressed.
Stage 4 – Data-Driven Culture
Stage 4 marks a significant shift in data accessibility and cultural transformation. Digital data is more widely available than ever. It is possible that some data silos may persist. This stage demonstrates that data initiatives are taking root, fostering a workplace where employees use data to support their decisions.
At this stage, the data ecosystem is robust enough to support value creation through data-driven decision-making. However, there may still be room for improvement in data integration and data quality. Decisions are typically reactive but are now backed by evidence, promoting a culture that emphasizes the importance of continuous data use and collaboration. This is where sites start aligning their data storage solutions to ensure broader access and integration with different data types, preparing for more advanced processes. This is the point where AI and ML integrations can join the conversation.
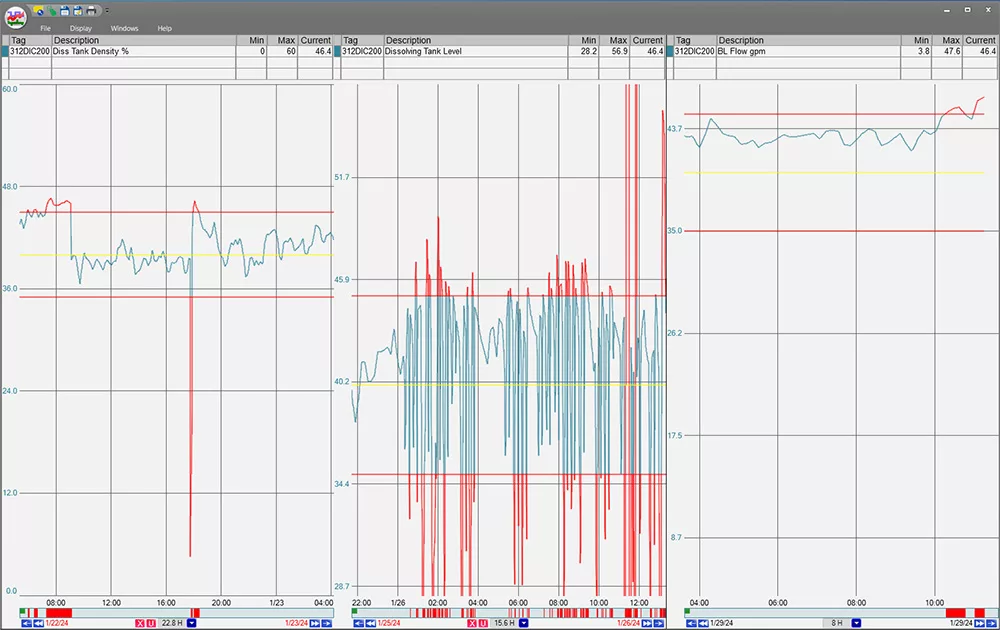
Data silos are elimiated with PARCview and users can trend process data next to lab data. All in one place. Enable limits and get alarms when key values go out of spec.
Stage 5 – Data-Driven is in the DNA
At Stage 5, the data ecosystem has reached a mature state, characterized by a universal data platform and the elimination of data silos. There is comprehensive access across the entire organization. The organization fosters seamless data integration and ensures that data quality is maintained at the highest level. This stage supports proactive, real-time decision-making, paving the way for AI and ML to optimize manufacturing processes.
Advanced analysis and predictive models, such as predictive analytics and preventive maintenance, become part of daily operations. With shared data fully implemented and a cohesive data storage strategy in place, the organization is well-positioned for artificial intelligence-driven insights and innovation. If they have not started looking into AI and ML options, now is the time.
Improve Your Data Ecosystem- Preparing to Implement AI the Right Way
If your site currently falls short of level 4 or level 5 on the digital transformation roadmap, significant changes are necessary before implementing AI. Enhancing a manufacturing facility’s data ecosystem requires a thorough understanding of your starting point on this roadmap. Unfortunately, it will not be as simple as purchasing equipment or software with a simple checklist. Building a mature digital ecosystem involves fostering a data-driven culture integrating digital technologies into business processes.
This transformation takes time and must be approached methodically. Rushing from level 1 to an artificial intelligence-ready state can lead to gaps that hinder long-term success. Below are practical strategies to get employees engaged with data. To help foster a culture of data-driven insights and prepare all aspects of your site for the AI journey.
Stage 1 – No Data, No Time
At Stage 1, you’re essentially starting from scratch, meaning significant investments in time and capital will be needed to establish a foundational digital ecosystem. This initial step involves integrating programmable logic controllers (PLCs) and sensors into your processes. These tools capture raw data from various points across your manufacturing line, providing a real-time understanding of process conditions.
Establishing this baseline knowledge is crucial for enabling future data collection, analysis, and the implementation of advanced digital technologies. Without this foundation, any attempt to use AI for business processes or predictive analysis will be ineffective.
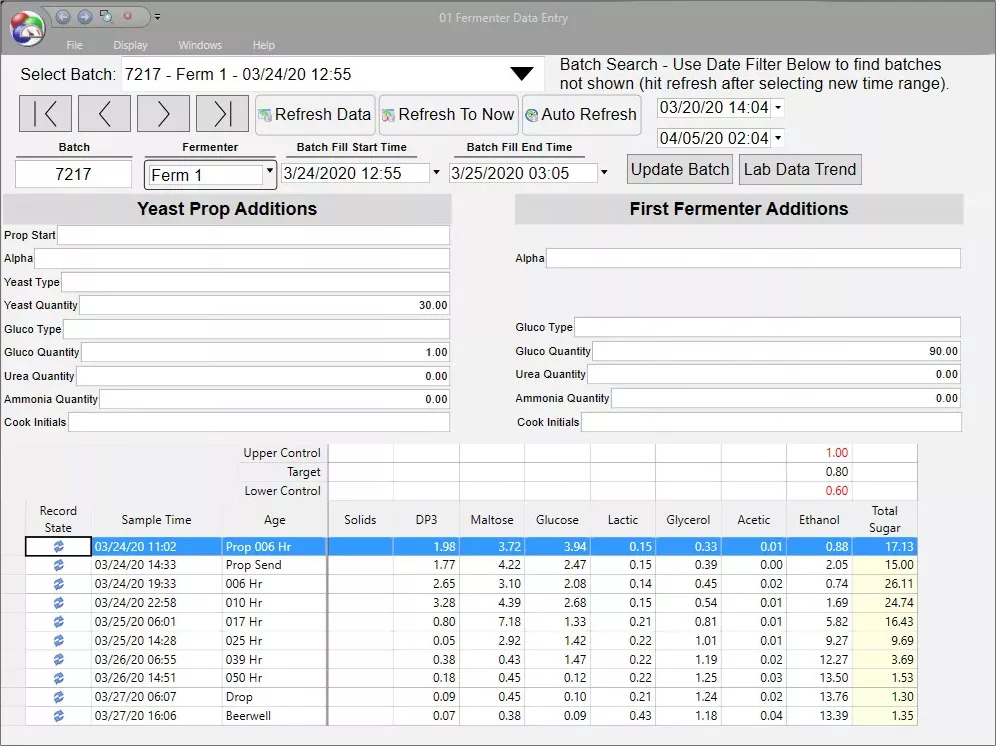
If you have a lot of manual data, consider moving it out of Excel or Paper logs to a LIMS or system where it can be saved and backed up into a database.
Stage 2 – Some Data, Still Little Time
With PLCs and sensors in place, the next step is to implement a data historian. A robust historian like dataPARC can store data systematically and facilitate data-driven insights by consolidating information for easier access. dataPARC’s tag-based pricing model allows you to start small and expand as your digital ecosystem grows. At this stage, data initiatives should include encouraging employees to access stored data regularly and understand its value for decision-making. By emphasizing consistent data usage, you’re laying the groundwork for more comprehensive data integration down the line.
Stage 3 – Reflective Decision-Making
To advance beyond Stage 3, sites must actively utilize their existing data ecosystems to drive better business processes. This includes expanding the amount of data stored in a data historian and ensuring that employees have visibility and access to this information. A reliable data visualization software, such as dataPARC’s PARCview, can be a game-changer at this level. It enables users to build trends, generate visual dashboards, and monitor key metrics, fostering confidence in data use across teams. The aim here is to transform raw data into actionable insights that inform decisions and improve efficiency.
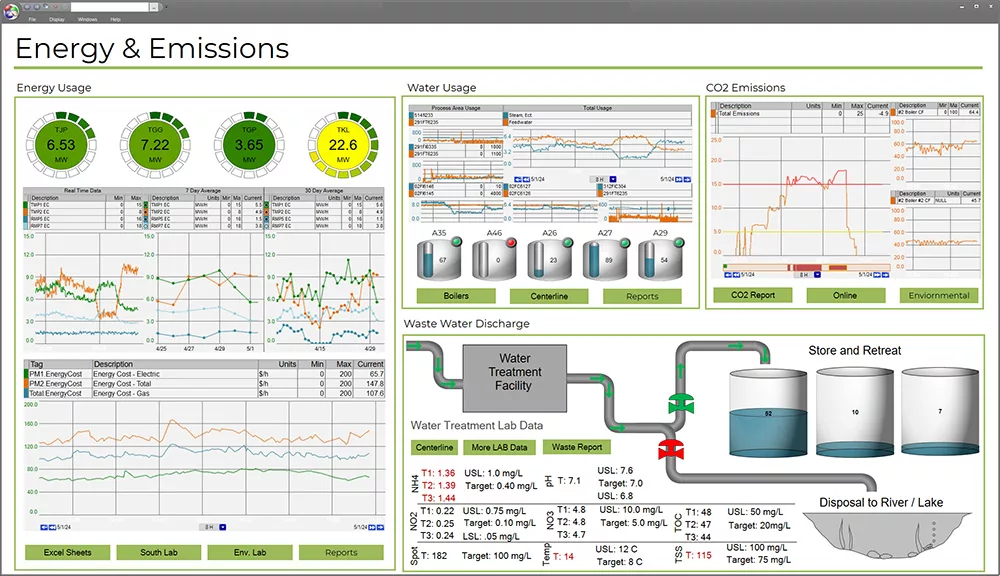
Real-time production monitoring and getting data in front of those who need it is critical for building a data-driven culture.
Stage 4 – Data-Driven Culture
Transitioning from Stage 4 to Stage 5 involves more than just technical upgrades; it requires embedding data into the company’s culture. To achieve this, sites should expand their data integration capabilities beyond just processing data.
PARCview, an industrial data platform, allows users to incorporate various data types, including Manufacturing Execution Systems (MES), Laboratory Information Management Systems (LIMS), data from other historians, and various external data sources. Creating this interconnected digital ecosystem ensures that data silos are eliminated, ensuring compliance, and employees can seamlessly access comprehensive, real-time data. This shift enables quicker, more effective decision-making and empowers a data-driven culture that supports business goals.
Stage 5 – Data-Driven is in the DNA
At Stage 5, your site has reached full digital maturity with a comprehensive, unified data platform that serves as a single source of truth. All data types are integrated into a cohesive digital ecosystem, and there are no remaining data silos. At this level, access to data is widespread, facilitating a collaborative environment where advanced digital technologies, such as AI and ML, can be fully leveraged. At this point, your site is ready to research and implement specific AI and ML software solutions tailored to your operational goals.
With a strong data foundation, these tools can optimize business processes through predictive maintenance, data protection, soft sensors, and continuous improvement. Your data ecosystem is now positioned to support advanced data initiatives, drive innovation, and create substantial value for your operations.
Other Considerations Before Implementing Data Ecosystem for AI
Before integrating AI into your manufacturing processes, it’s essential to address several critical considerations. Throughout your digital transformation journey, many steps will reveal gaps in your current data ecosystem, such as areas where key metrics are not adequately measured or monitored. No amount of artificial intelligence or advanced digital technologies can compensate for fundamental data problems. Issues like incomplete data collection, poor data quality, and disjointed data storage can severely limit the effectiveness of AI, leading to suboptimal outcomes and wasted investments.
1. Identifying Gaps in Data Collection and Quality
A successful AI strategy begins with high-quality data. Evaluate your current data collection practices to identify any gaps that might hinder AI implementation. Ensure that all critical process metrics are measured consistently and accurately. Conduct audits to assess data quality and standardize data formats, making them AI-ready. Without this foundational step, AI models may yield unreliable or skewed results, diminishing their value in your business processes.
2. Integrating AI as an Extension of SME Expertise
AI should be viewed as an enhancement to the work that subject matter experts (SMEs) are already performing rather than as a replacement for foundational tasks. SMEs provide invaluable context and insights that AI algorithms need for effective deployment. AI can automate routine data analysis, identify trends, detect anomalies, and provide actionable suggestions that might not be immediately evident to human analysts. This collaboration ensures that AI augments human expertise, enabling more informed, data-driven decisions.
3. Ensuring Data Integration Across Systems
For AI to function effectively, your data ecosystem must support seamless data integration. AI applications rely on comprehensive, real-time data from multiple sources to generate meaningful insights. A unified data platform that consolidates process data, MES, LIMS, and other data sources is vital for eliminating silos and ensuring consistent data quality. Investing in tools that facilitate smooth data integration and sharing will prepare your digital ecosystem for the demands of AI.
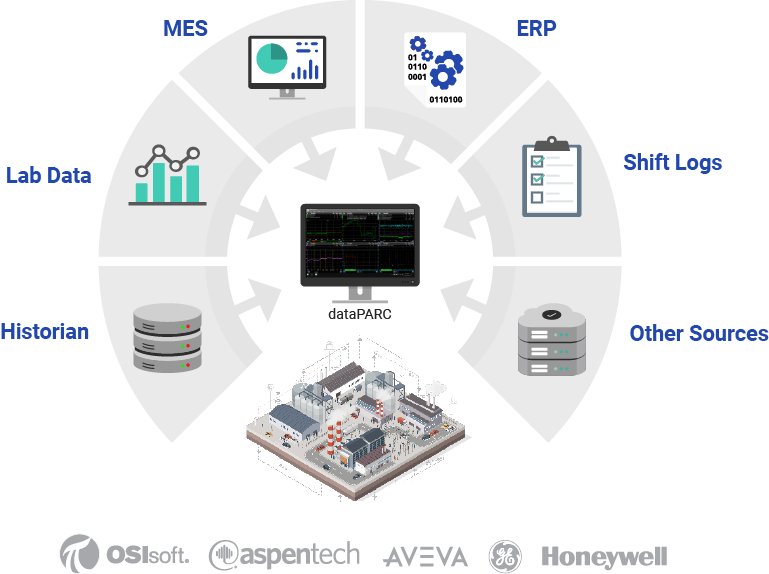
Having a single source of truth through data integration helps keep everyone on the same page.
4. Aligning AI with Business Processes
AI should be incorporated as part of a cohesive strategy that aligns with the overall business goals of stakeholders within the organization. When AI is implemented with clear objectives—such as enhancing production efficiency, reducing downtime, or improving product quality—it becomes an invaluable part of the business process. Ensure that AI initiatives are mapped to your operational KPIs and contribute to the overarching mission of value creation and continuous improvement.
5. Building a Data-Driven Culture
A crucial component of AI readiness is fostering a data-driven culture. This means training employees to use data as part of their day-to-day operations and empowering them with the skills needed to leverage AI tools effectively. Workshops, training sessions, and change management strategies can help build confidence in using digital technologies and encourage collaboration between IT/OT teams, SMEs, and management. The goal is to create an environment where data-driven insights are integral to decision-making processes.
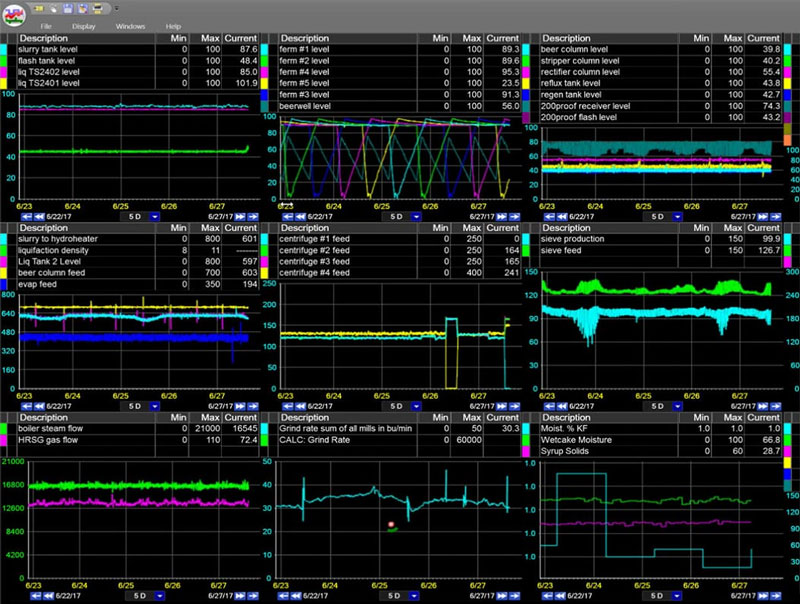
Check out dataPARC’s real-time process data analytics tools & see how better data can lead to better decisions.
6. Monitoring and Iterating AI Implementations
Implementing AI is not a one-time event but an ongoing journey. Continuous monitoring of AI performance, gathering user feedback, and iterating on AI models and processes are crucial for long-term success. This iterative approach ensures that AI solutions remain effective and adaptable to evolving business needs and challenges. Regular updates and evaluations can help refine AI outputs, making them even more valuable over time.
By addressing these considerations, your site can be better prepared to incorporate AI in a way that maximizes value, improves efficiency, and supports sustainable growth. Remember, AI should complement your existing business processes and be an extension of the data initiatives your site has built over time. Ensuring that your digital ecosystem is robust and well-integrated will pave the way for a smooth and effective transition into AI-powered manufacturing services.
Final Thoughts: Readiness of Data Ecosystem for AI Starts with a Strong Data Foundation
Before embracing AI technologies, manufacturers must ensure their sites already operate with a strong data foundation. If your teams are not currently using data for daily operations, troubleshooting, process optimization, and strategic planning, they are not prepared to use AI tools effectively. Successful AI implementation relies on a culture where data is the backbone of decision-making and continuous improvement.
AI should be viewed as an extension of existing data-driven practices rather than a solution that replaces them. Sites that have already integrated data usage into their workflow are better equipped to adopt AI, as their teams are accustomed to leveraging data for insights and action. This readiness helps AI tools become more than just technological add-ons; they become powerful contributors to optimizing operations, predicting maintenance needs, and driving strategic growth.
Ultimately, the journey to AI should start with ensuring that your data ecosystem is mature, integrated, and capable of supporting advanced analytics. Only then can AI serve as a valuable tool that empowers your site to move from reactive responses to proactive decision-making and continuous value creation.