Both established operational data historians and newer open-source platforms continue to evolve and add new value to business, but the significant domain expertise now embedded within data historian platforms should not be overlooked.
Time-series databases specialize in collecting, contextualizing, and making sensor-based data available. In general, two classes of time-series databases have emerged: well-established operational data infrastructures (operational, or data historians), and newer open source time-series databases.
Data Historian vs. Time Series Database
Functionally, at a high level, both classes of time-series databases perform the same task of capturing and serving up machine and operational data. The differences revolve around various formats of data, features, capabilities, and relative ease of use.
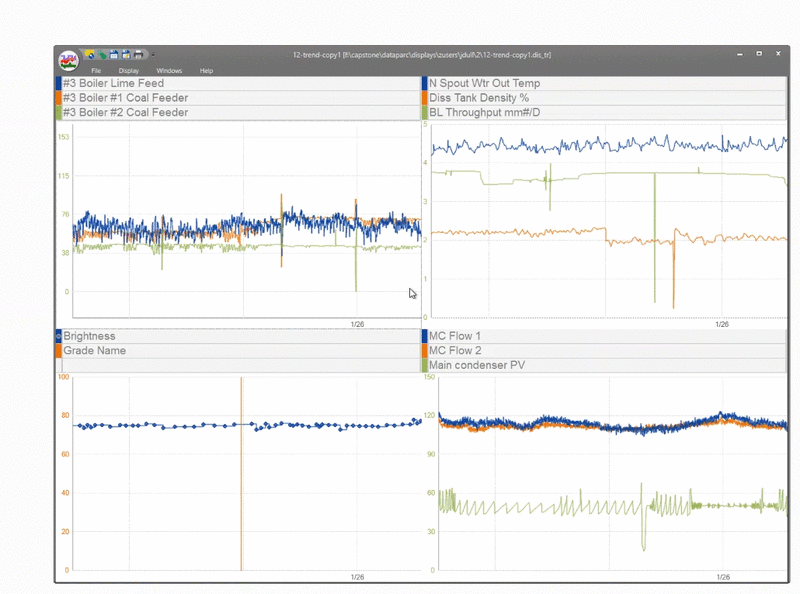
Time-series databases and data historians, like dataPARC’s PARCserver Historian, capture and return time series data for trending and analysis.
Benefits of a Data Historian
Most established data historian solutions can be integrated into operations and begin collecting data relatively quickly. The industrial world’s versions of commercial off-the-shelf (COTS) software, such as established data historian platforms, are designed to make it easier to access, store, and share real-time operational data securely within a company or across an ecosystem. This is particularly crucial in scenarios of low bandwidth data communications, ensuring efficient collection and dissemination of production data, whether it’s generated by machinery, production processes, or other vital operational components.
While, in the past, industrial data was primarily consumed by engineers and maintenance crews, this data is increasingly being used by IT due to companies accelerating their IT/OT convergence initiatives. These departments, as well as financial departments, insurance companies, downstream and upstream suppliers, equipment providers selling add-on monitoring services, and others, need to utilize the same data. While the associated security mechanisms for industrial internet were already relatively sophisticated, they are evolving to become even more secure.
Another major strength of established data historians is that they were purpose-built and have evolved to be able to efficiently store and manage time-series data from industrial operations. As a result, they are better equipped to optimize production, reduce energy consumption, implement predictive maintenance strategies to prevent unscheduled downtime, and enhance safety. The shift from using the term “data historian” to “data infrastructure” is intended to convey the value of compatibility and ease-of-use of this data-driven and data generated approach.
Searching for a data historian? dataPARC’s PARCserver Historian utilizes hundreds of OPC and custom servers to interface with your automation layer.
What about Time Series Databases?
In contrast, flexibility and a lower upfront purchase cost are the strong suits for the newer open source products. Not surprisingly, these newer tools were initially adopted by financial companies (which often have sophisticated in-house development teams) or for specific projects where scalability, ease-of-use, and the ability to handle real-time data are not as critical.
Since these new systems were somewhat less proven in terms of performance, security, and applications, users were likely to experiment with them for tasks in which safety, lost production, or quality are less critical.
In industrial data management, “standard database systems” represent the enduring pillars for storing historical data generated during manufacturing processes. Unlike newer open-source alternatives, these systems have been the backbone of industrial data storage infrastructure, offering a consistent method to capture and preserve data points from diverse sources. Their proven ability to handle vast amounts of continuous data makes them indispensable in manufacturing, ensuring production plants have a reliable foundation for comprehensive record-keeping.
While some of the newer open source time series databases are starting to build the kind of data management capabilities already typically available in a mature operational data historian, they are not likely to completely replace operational data infrastructures in the foreseeable future.
Industrial organizations should use caution before leaping into newer open source technologies. They should carefully evaluate the potential consequences in terms of development time for applications, security, costs to maintain and update, regulatory requirements and their ability to align, integrate or co-exist with other technologies. It is important to understand operational processes and the domain expertise and applications that are already built-into an established operational data infrastructure.
These standard systems shine in environments dealing with data from multiple sources, for example seamlessly integrating inputs from machinery sensors to manual sources. Versatility is a hallmark, allowing organizations to centralize diverse data points.
The inherent consistency in their approach ensures uniform treatment of every data point, a critical factor for accurate analysis and reporting in industrial settings. In essence, standard database systems stand as the bedrock of effective manufacturing data management software, providing a reliable, consistent, and versatile framework for robust decision-making processes.
Why use a Data Historian?
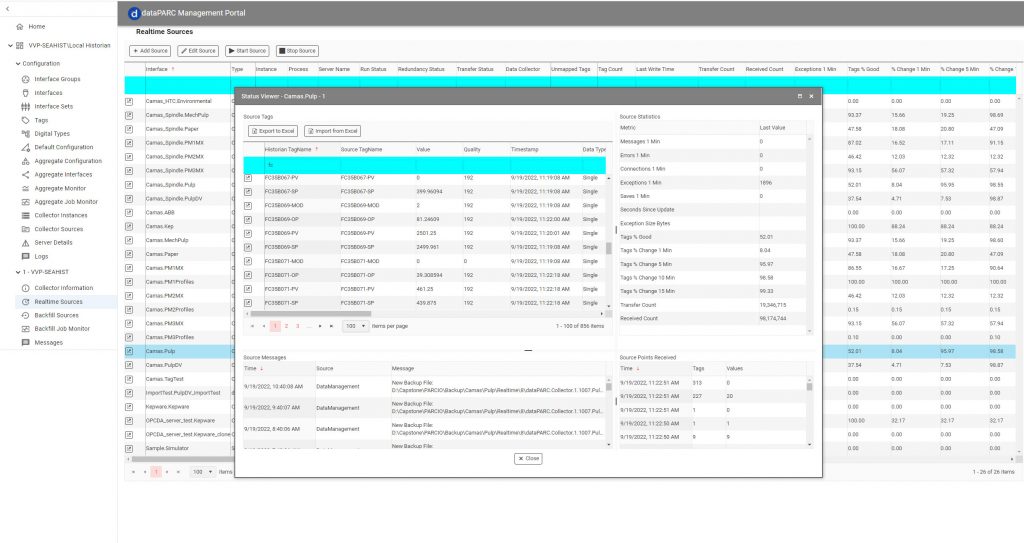
Typical connection management and config area from an enterprise data historian.
When choosing between data historians and open source time-series databases, many issues need to be considered and carefully evaluated within a company’s overall digital transformation process. These include type of data, speed of accessing data, industry- and application-specific requirements, legacy systems, and potential compatibility with newly emerging technologies.
According to recent data due to the process industry consulting organization ARC Advisory Group, modern data historians and advanced data storage infrastructures will be key enablers for the digital transformation of industry. Industrial organizations should give serious consideration when investing in modern operational data historians and data platforms designed for industrial processes.
11 Things to Consider When Selecting Modern Data Historians for Manufacturing Operations & Data Analysis:
1. Data Quality
When considering data historians for manufacturing operations and data analysis, data quality stands as a paramount consideration. The chosen system should exhibit an ability to not only store data but also ingest, cleanse, and validate data. A crucial aspect is ensuring that data is recorded accurately and represents the intended information.
For instance, in scenarios where calibration data is involved, the historian should discern and appropriately handle this information to prevent skewing averages. Similarly, when operators or maintenance personnel engage with controllers or override alarms, the historian must capture and store this data accurately, reflecting the true state of the system.
Therefore, evaluating the system’s capacity to maintain data integrity in diverse operational scenarios is vital in ensuring the reliability of the recorded information.
2. Contextualized Data
When dealing with asset and process models based on years of experience integrating, storing, and accessing industrial process data and its metadata, it’s important to be able to contextualize data easily. A key attribute is the ability to combine different data types and different data sources.
Can the process historian combine data from spreadsheets and different relational databases, or multiple sources, precisely synchronize timestamps, and be able to make sense of it?
3. High Frequency/High Volume Data
It’s also important to be able to manage large volumes of high-frequency, high-volume data based on the process requirements, and expand and scale as needed. Increasingly, this includes edge and cloud capabilities.
As companies progress along their digital transformation journey, the escalating demand for data becomes increasingly evident, with discussions on big data becoming a daily occurrence. This is precisely where the significance of managing high-frequency data comes into play.
A robust data historian is essential, capable of efficiently processing high speed data collection, ensuring seamless management and scalability to meet the expanding needs and requirements of evolving manufacturing processes.
4. Real-Time Accessibility
Accessing your time series data, event data, and process data is pivotal for optimizing industrial processes. Quick and seamless access to this information is instrumental in troubleshooting upsets swiftly, reducing downtime, and ensuring that insights from data are delivered at the speed of thought.
Modern data historians, designed for real-time accessibility, can empower organizations to not only run their processes more efficiently but also proactively prevent abnormal software behavior, providing significant insights and value to the overall operational framework.
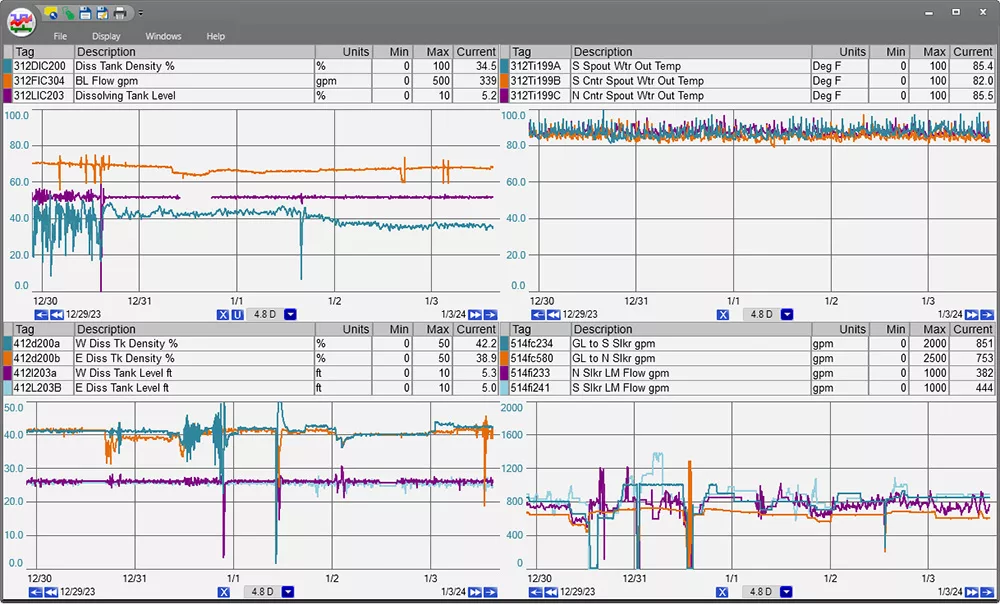
PARCview’s multi-trends allow for users to see large amounts of data on one screen.
5. Data Compression
Data compression, implemented through specialized algorithms, is a crucial aspect of data historians. These algorithms enable efficient compression of data while retaining the ability to reproduce trends when necessary.
However, it’s imperative to emphasize that data compression should not compromise data integrity or alter the fundamental shape of your process data. In the compression process, it’s essential to avoid the loss of important aggregates.
Different data historians employ varied approaches to data compression, underscoring the importance of understanding what best aligns with the specific requirements of your site. Selecting a data historian that ensures optimal data compression without sacrificing integrity is paramount for accurate and meaningful historical data analysis.
6. Sequence of Events
Sequence of Events (SOE) capability in a data historian is instrumental in providing a detailed and accurate account of occurrences within operations plant data, or a production process. This feature allows users to precisely reproduce events, offering a comprehensive understanding of the chronological order in which they occurred.
7. Statistical Analytics
Incorporating statistical analytics capabilities within a time series data historian enhances its functionality by allowing for intricate spreadsheet-like calculations and complex regression analysis. This feature empowers users to extract meaningful insights from historical data, enabling more informed decision-making.
Moreover, in the era of real-time data, the ability of the historian to seamlessly stream data to third-party applications for advanced analytics, machine learning (ML), or artificial intelligence (AI) is crucial. This not only facilitates real-time analytics but also opens the door to generating dynamic reports based on the outcomes of statistical analyses.
The integration of real-time data into statistical analytics not only improves the accuracy and relevance of the analyses but also ensures that decision-makers have access to the most up-to-date information for timely and effective actions.
8. Visualization
Effortlessly creating and tailoring digital dashboards with enhanced situational awareness is crucial for seamless manufacturing processes. Different individuals, from the operations floor to the corporate level, often necessitate varied perspectives of the data.
A versatile visualization tool that offers multiple options, such as trending, dashboards, and report generation, proves invaluable in meeting the diverse needs of every worker. Whether through trends, dashboards, or detailed reports, a comprehensive visualization tool ensures that users can interpret and analyze production data in the most effective and meaningful manner tailored to their specific requirements.
9. Connectability
The capability to seamlessly connect to multiple data sources together is essential. Thus, collecting and storing data is necessary, as well as integrating with various control systems is also key. While the OPC standard is widely used and reliable, it might not be universally suitable for all applications.
Although the process of establishing these connections can be time-consuming, leveraging specialized connectors proves beneficial, ensuring a link between the data historian and the multitude of data sources from within the manufacturing ecosystem.
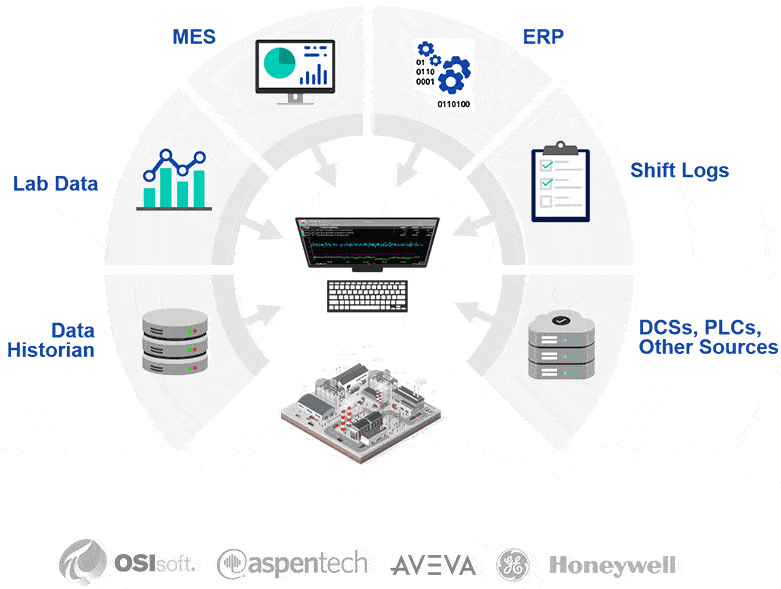
Integrate your data from any source with PARCview.
10. Timestamp Synchronization
Timestamp synchronization helps ensure timestamp accuracy, in turn ensuring data accuracy. This is pivotal for ensuring accurate alignment between the data in standard database system and its associated metadata, whether the data storage is on-premises or in the cloud.
Precise timestamp synchronization is especially critical when you collect data from instruments distributed across different geographical locations or sourced from various systems. No concern about mismatched system clocks. A time synchronization mechanism guarantees that the data accurately reflects the chronological order of events, providing users with a reliable foundation for analysis and decision-making.
11. Partner Ecosphere
The significance of a partner ecosystem cannot be overstated in the context of data historians. An expansive partner ecosystem can significantly enhance the functionality and value of the data historian by providing users with the flexibility to integrate purpose-built vertical applications seamlessly.
This integration goes beyond the core infrastructure, allowing users to leverage specialized tools and applications tailored to their specific industry or operational requirements.
The collaborative efforts within a rich partner ecosystem can result in the development of innovative solutions that address unique challenges in manufacturing processes. Additionally, a well-established partner ecosystem ensures continuous support, updates, and a steady influx of new capabilities, contributing to the longevity and adaptability of the data historian solution.
What’s Next for your Manufacturing Data
Rather than compete head on, it’s likely that the established historian/data infrastructures and open-source time-series relational databases will continue to co-exist in the coming years. As the open-source time series database companies progressively add distinguishing features to their products over time, it will be interesting to observe whether they lose some of their open-source characteristics. To a certain extent, we previously saw this dynamic play out in the Linux world.