The most important differences between relational databases, time-series databases, and data lakes and other data sources are the ability to handle time-stamped process data and ensure data integrity.
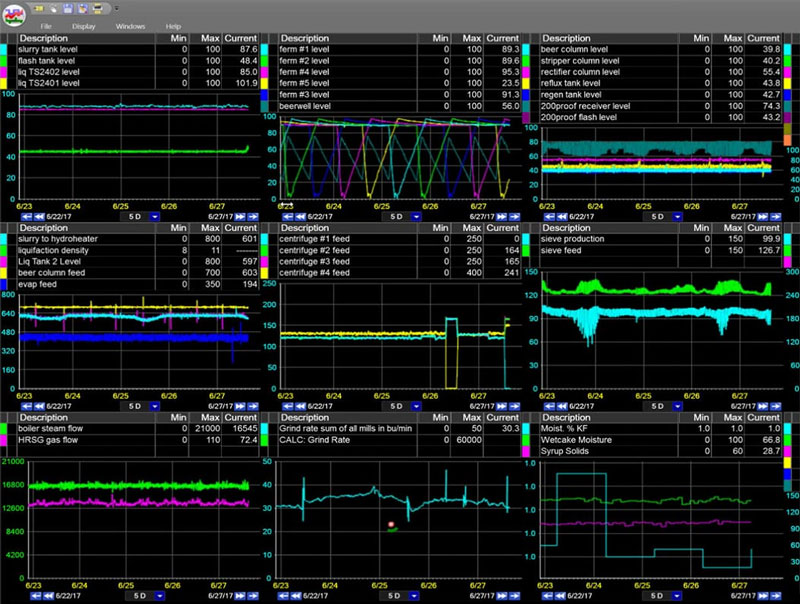
Enterprise data historian functionality at a fraction of the cost. Industrial time series data collection & analytics tools.
The Manufacturing Database Battle
The most important differences between relational databases, time-series databases, and data lakes and other data sources are the ability to handle time-stamped process data and ensure data integrity.
This is relevant because the primary job of the data management technology is to:
- Accurately capture a broad array of data streams
- Deal with very fast process data
- Align time stamps
- Ensure the quality and integrity of the data
- Ensure cybersecurity
- Serve up these data streams in a coherent, contextualized way for operational personnel
Time-Series Databases
Digital technologies and sensor-based data are fueling everything from advanced analytics, artificial intelligence and machine learning to augmented and virtual reality models. Sensor-based data is not easily handled by traditional relational databases. As a result, time-series databases have been on the rise and, according to ARC Advisory Group research, this market is growing much more rapidly than traditional relational databases.
While relational databases are designed to structure data in rows and columns, a time-series database or infrastructure aligns sensor data with time as the primary index.
Time-series databases specialize in collecting, contextualizing, and making sensor-based data available. In general, two classes of time-series databases have emerged: well-established operational data infrastructures (operational, or data historians), and newer open source time-series databases.
To gain maximum value from sensor data from operational machines, data must be handled relative to its chronology or time stamp. Because the time stamp may reflect either the time when the sensor made the measurement, or the time when the measurement was stored in the historian (depending upon the data source), it is important to distinguish between the two.
Searching for a data historian? dataPARC’s PARCserver Historian utilizes hundreds of OPC and custom servers to interface with your automation layer.
Relational Databases
Time series data technologies – whether open-source databases or established historians – are built for real-time data. Relational databases, in contrast, are built to highlight relationships, including the metadata attached to the measurement (alarm limits, control limits, customer spend, bounce rate, geographic distribution between different data points, etc.). Relational technologies can be applied to time series data, but this requires substantial amounts of data preparation and cleaning and can make data quality, governance, and context at scale difficult.
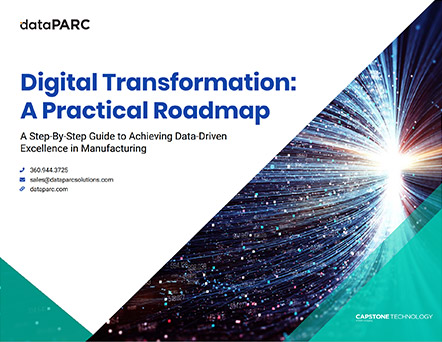
Integrating manufacturing data at your plant? Let our Digital Transformation Roadmap guide your way.
Data Lakes
Data lakes, meanwhile, score well on scalability and cost-per-GB, but poorly on data access and usability. Not surprisingly, while data lakes have the most volume of data, they typically have fewer users. As with time series technologies, the market will decide the time in which and how these different technologies get used.
Looking Ahead
Digital technologies and sensor-based data are fueling everything from advanced analytics, artificial intelligence and machine learning to augmented and virtual reality models. The fourth industrial revolution, or Industrie 4.0, along with major market disruptions, such as the pandemic driving sustainability, and operational resilience initiatives, has led to a great acceleration of digital transformation and exponential changes in industrial operations and manufacturing taking place.